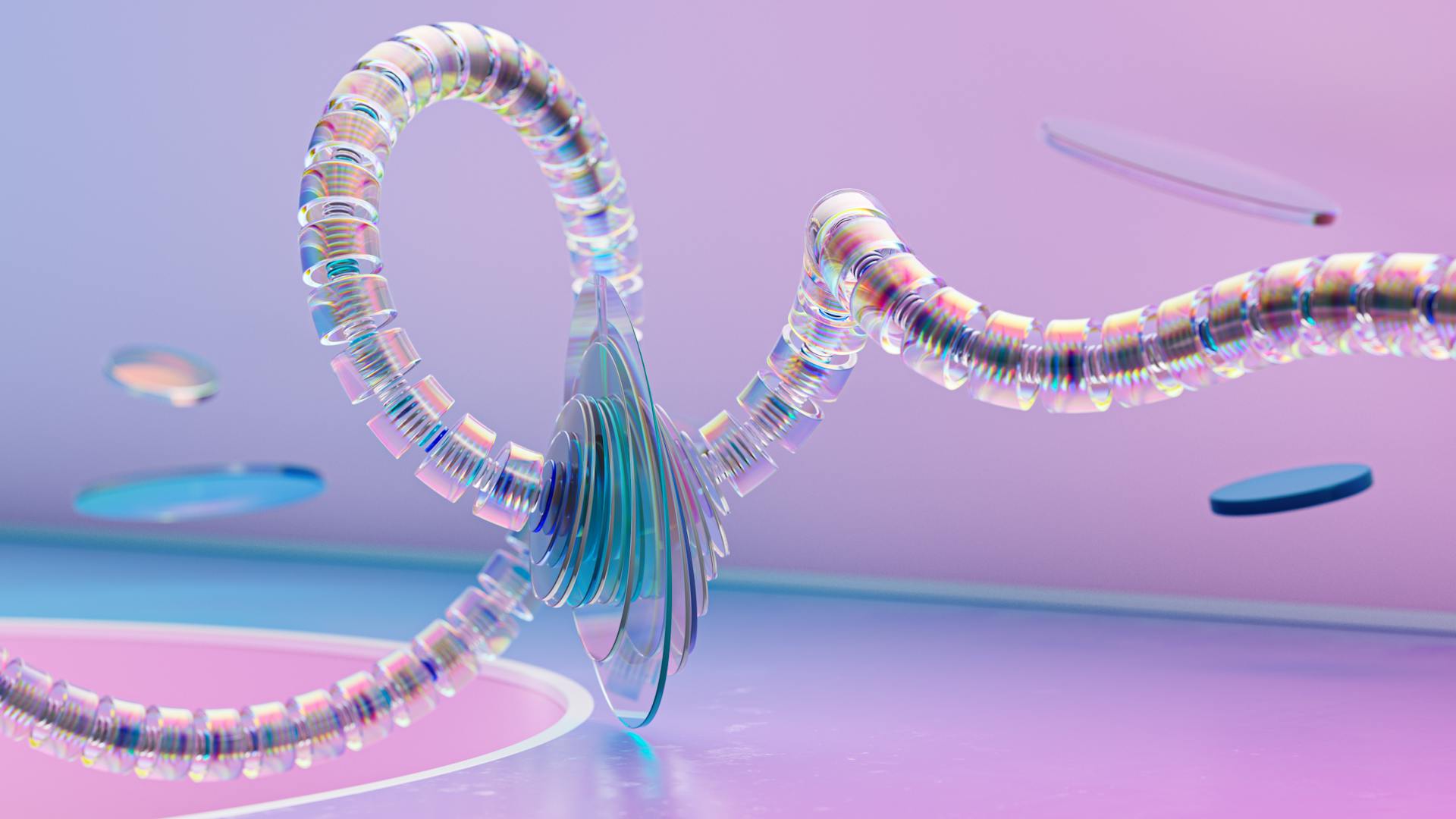
A data lake is a centralized repository that stores raw, unprocessed data in its native format, allowing for flexible and cost-effective data management. This approach is particularly beneficial for organizations with large amounts of data from various sources.
Data lakes can store data of any size and format, making them ideal for handling big data. According to Data Lake Inc, a data lake can store up to 100 petabytes of data, which is equivalent to about 100 million gigabytes.
One of the primary challenges of a data lake is data governance, which refers to the process of managing and maintaining the quality and integrity of the data. Data Lake Inc notes that data governance is crucial for ensuring that the data stored in a data lake is accurate, complete, and consistent.
Data lakes can be complex to manage, but with the right tools and expertise, they can provide numerous benefits, including improved data quality, reduced data silos, and enhanced business insights.
What is a Data Lake
A data lake is a centralized repository that stores data of many types at enterprise scale. It's like a big container that holds all your data in one place.
Data lakes are designed to handle large amounts of data, including unstructured, semi-structured, and structured data. They can store trillions of files, with each file being over a petabyte in size, which is 200x larger than other cloud stores.
Data lakes are different from data swamps, which lack proper data governance practices and result in chaotic and unusable data. To prevent a data lake from turning into a data swamp, strong governance and metadata management are essential.
Here are some key characteristics of a data lake:
- Massively scalable
- No limits to the size of data
- Ability to run massively parallel analytics
Data lakes are particularly useful for storing historical data, which can have many uses, including error recovery, validation of data systems or flows, and exploratory analysis.
Swamp Definition
A data swamp is essentially a poorly managed data repository where data is ingested without proper governance, leading to disorganized, undocumented, and useless data.
Data swamps happen when a data lake gets abused, and it's a collection point for miscellaneous data that no longer has any structure.
In a data swamp, data is ingested without proper governance, resulting in data that is disorganized, undocumented, and essentially useless.
This makes it difficult to retrieve, analyze, and leverage data, ultimately impeding business decision-making.
A data swamp lacks proper data governance practices, which ensures data quality and usability in a data lake.
Implementing strong governance and metadata management can prevent a data lake from turning into a data swamp.
Data swamps can quickly become dumping grounds where data becomes harder to find, evaluate, or use, making it essential to keep your data lake clean and organized.
From Silos to Warehouses
Data silos were once the norm for managing different types of data. However, they couldn't lead to meaningful insights and couldn't make the most of data for organizations seeking to modernize their data to the cloud.
In the early internet era, data silos were used to manage several different types of data, but they were not organized together in a way that led to meaningful insights. This is especially true with the immense amount of data generated by today's organizations.
A data warehouse is an enterprise infrastructure that allows businesses to bring together and access various structured data sources. These data sources include the kind that were historically managed with different silos.
Data warehouses have a harder time understanding unstructured data, which includes clicks on social media, input from IoT devices, and user activity on websites. This type of data can be valuable to commerce and business, but it is more difficult to store and track than structured data.
In contrast, data lakes store data from many sources, including unstructured sources like log data, IoT sensors, and social media feeds. A data lake platform is basically a collection of raw data assets that come from an organization's business operations and other sources, both internal and external.
Here are some key differences between data warehouses and data lakes:
In the old world of expensive, bulky database storage, storing both historical and current analytics data seemed out of the question. But in modern data lakes, with almost unlimited low-cost storage, and elastic scalability, it becomes possible.
Benefits of a Data Lake
A data lake is a game-changer for businesses, offering numerous benefits that can help you unlock the full potential of your data.
Storing vast amounts of raw, unstructured data in a cost-effective manner is one of the key benefits of a data lake. This allows organizations to derive more value from their data, enabling data-driven decision-making.
Data lakes empower business users to find relevant information faster, regardless of sources or locations. This then enables more effective analyses, deeper cross-organization collaboration, and faster, more informed decision-making.
A data lake gives businesses a common repository to collect and store data, streamlined usage from a single source, and access to the raw data necessary for today's advanced analytics and artificial intelligence (AI) needs.
Here are some of the key benefits of a data lake:
- Resource reduction: Being able to store any kind of data means resource savings at no loss of value.
- Organization-wide accessibility: Data lakes provide a way around rigid silos and bureaucratic boundaries between business processes.
- Performance efficiency: Data lakes do not require data to be defined by schemas.
Data lakes are also a cost-effective solution to run big data workloads. You can choose between on-demand clusters or a pay-per-job model when data is processed, and you never pay for more than you need.
Best Practices for Managing a Data Lake
Managing a data lake effectively requires adherence to best practices that ensure data integrity, accessibility, and security.
Implementing robust data governance policies is crucial to prevent data swamps and ensure data quality. According to Example 1, organizations should prioritize user access controls to protect sensitive information while enabling data discovery for analysts.
Maintaining comprehensive metadata in a data catalog is essential for data governance, as it provides visibility into the data inventory and answers questions about business context, lineage, and value. This is facilitated by metadata management, as explained in Example 5.
Automated data quality checks can help streamline operations and prevent data governance complexities. By following best practices, businesses can prevent data swamps and maximize the value derived from their data lakes.
Effective data governance addresses key elements such as data quality, security, compliance, and usage policies. This is critical for the successful management of data lakes and lakehouses, as highlighted in Examples 7 and 9.
Establishing clear roles and responsibilities for data stewardship ensures that data remains reliable and trustworthy. By prioritizing data governance, organizations can harness the full potential of their data assets while maintaining compliance and safeguarding sensitive information.
Challenges of Managing a Data Lake
Managing a data lake can be a daunting task. A data lake without proper oversight can become unwieldy, leading to inefficiencies and potential compliance risks.
Data governance complexities, security vulnerabilities, and difficulties in maintaining data quality are common issues that organizations must navigate. Without proper oversight, data lakes can become a data swamp, a chaotic, unorganized mess of data that's difficult to access, analyze, and use effectively.
Here are some key challenges to consider:
- Data governance complexities
- Security vulnerabilities
- Difficulties in maintaining data quality
By proactively identifying and addressing these challenges, businesses can create a more sustainable data lake environment that delivers ongoing value and supports strategic objectives.
A Management Nightmare
A data lake can quickly become a management nightmare if not properly managed. This chaotic mess of unorganized data is difficult to access, analyze, and use effectively.
The dangers of a data swamp, a common problem for businesses, include difficulties in accessing, analyzing, and using the data. It's a chaotic, unorganized mess that can lead to inefficiencies and potential compliance risks.
Proper oversight is crucial to avoid turning a data lake into a data swamp. Without it, data lakes can become unwieldy, leading to inefficiencies and potential compliance risks.
A data lake can become a data swamp due to design mistakes, stale data, or uninformed users and lack of regular access. Businesses should anticipate several challenges when building a data lake to avoid this fate.
To avoid a data swamp, businesses should:
- Set business priorities and assess what data is useful to store
- Designate use cases and end users to ensure data is accurate and fit for purpose
- Commit to good communication to maintain focus and ensure stakeholders are aware of how to use the data
- Establish a robust data ingestion process to get uncorrupted raw data into storage
A lack of automation can also contribute to a data lake becoming a data swamp. Automated data maintenance and cataloging practices are essential to keeping pace with a growing data lake.
The volume of metadata in a cloud data lake increases proportionally with the amount of data. This makes metadata management critical when building a data-driven business.
Cost Overruns
Cost overruns can be a significant challenge when managing a data lake. Organizations often presume that moving to the cloud will automatically lower ongoing costs.
Cloud environments can help eliminate data center expenses, but it's essential to apply a flexible, data consumption-based pricing model with a data lake. This model ensures that each user and team pays only for the precise compute and storage resources they use.
AutoDM, or autonomous data management, can help ease bottlenecks by using metadata, automation, and AI to standardize and accelerate data delivery with minimal human intervention.
Data Lake Architecture
A data lake can have various types of physical architectures because it can be implemented using many different technologies. However, there are three main principles that differentiate a data lake from other big data storage methods.
All data is accepted to the data lake—it ingests and archives data from multiple sources, including structured, unstructured, raw, and processed data. This allows for a wide range of data types to be stored in the data lake.
Data is stored in its original form—after receiving the data from the source, the data is stored unconverted or with minimal treatment. This means that the data is not altered or processed in any way before it is stored.
Data transformed on demand—the data is transformed and structured according to the analysis requirements and queries being performed. This allows for dynamic querying and analysis of the data.
Most of the data in a data lake is unstructured and not designed to answer specific questions, but it is stored in a way that facilitates dynamic querying and analysis.
A data lake should have the following capabilities to keep it functional and make good use of its unstructured data:
- Data classification and data profiling—the data lake should make it possible to classify data, by data types, content, usage scenarios, and possible user groups.
- Conventions—the data lake should, as much as possible, enforce agreed file types and naming conventions.
- Data access—there should be a standardized data access process, used both by human users and integrated systems, which enables tracking of access and use of the data.
- Data catalog—the data lake should provide a data catalog that enables the search and retrieval of data according to a data type or usage scenario.
- Data protection—security controls, data encryption, and automatic monitoring must be in place, and alerts should be raised when unauthorized parties access the data, or when authorized users perform suspicious activities.
- Data governance—there should be clear policies, communicated to all relevant employees, about how to navigate and make use of the data lake, how to promote data quality, and ethical use of sensitive data.
Data lakes can be implemented using many different technologies, and can be hosted in both cloud and on-premises locations. This flexibility makes data lakes a popular choice for storing and managing large amounts of data.
Data Lake Security
Data Lake Security is a top priority for any organization considering a data lake solution. Enterprise-grade security, auditing, and support are built-in, backed by a 24/7 customer support team.
Microsoft's Data Lake is fully managed and supported, guaranteeing continuous operation and protecting your data assets with enterprise-grade security. Data is always encrypted, both in motion and at rest, using SSL and service or user-managed HSM-backed keys in Azure Key Vault.
Data governance and access control are also crucial aspects of data lake security. Data catalog solutions allow users to create a catalog of the data, specifying different types of data and the access controls and storage policies for each.
Access Control
Data lakes raise major security concerns because they contain many different types of data, some of which may be sensitive or have compliance requirements.
Permissions in data lakes are more fluid and difficult to set up because there are no database tables, making it harder to control who has access to the data.
Data catalog solutions can be used to create a catalog of the data, specifying different types of data and the access controls and storage policies for each.
Data lakes store unstructured data and separate storage from computing, allowing you to store large amounts of data with a relatively small investment.
To control access to data in a lake, you need to set up permissions based on specific objects or metadata definitions, which can be a complex task.
Data governance tools can help solve the problem of access control in data lakes, making it easier to manage who has access to the data and what they can do with it.
Enterprise Grade Security and Support
Microsoft Data Lake offers enterprise-grade security, auditing, and support, backed by a robust SLA and 24/7 customer support.
Data Lake is fully managed and supported by Microsoft, giving you peace of mind knowing that your entire big data solution is in good hands.
Microsoft's team monitors your deployment, ensuring it runs continuously and continuously.
Data is always encrypted, both in motion using SSL and at rest using service or user-managed HSM-backed keys in Azure Key Vault.
Imperva provides activity monitoring for relational databases, data warehouses, and data lakes, generating real-time alerts on anomalous activity and policy violations.
Imperva's solution protects all cloud-based data stores to ensure compliance and preserve the agility and cost benefits you get from your cloud investments.
Data lakes raise major security concerns, but various governance tools can be used to control who has access to the data, such as data catalog solutions that allow users to create a catalog of the data.
Data is always encrypted, both in motion using SSL and at rest using service or user-managed HSM-backed keys in Azure Key Vault.
Data Lake Tools and Services
Data Lake offers a range of tools and services to make big data easy. With Data Lake, you can develop, debug, and optimize big data programs with ease, thanks to deep integration with Visual Studio, Eclipse, and IntelliJ.
Data Lake Analytics is a distributed analytics service that makes big data easy. It integrates seamlessly with your existing IT investments, including Azure Synapse Analytics, Power BI, and Data Factory.
Data engineers, DBAs, and data architects can use existing skills like SQL, Apache Hadoop, Apache Spark, R, Python, Java, and .NET to become productive on day one with Data Lake. This eliminates the need to rewrite code as you increase or decrease the size of the data stored or the amount of compute being spun up.
Data Lake Store can store trillions of files, where a single file can be greater than a petabyte in size, which is 200x larger than other cloud stores. This means you can analyze all of your data in a single place with no artificial constraints.
Data Lake provides a holistic data quality experience that delivers trusted, timely, and relevant information to support any analytics or AI/ML initiatives. This is critical to cloud data lakes, especially when dealing with a variety of data.
You can integrate data from various disparate data sources at any latency with Data Lake, and rapidly develop extract, load, transform (ELT) or extract, transform, load (ETL) data flow.
Data Lake Use Cases
Data lakes can store and manage data from various sources and formats, making them a valuable tool for healthcare organizations that need to access patient data, research studies, and more.
In healthcare, a data lake can ingest unstructured data, such as patient heartbeat or oxygen levels, to provide insights for clinical and research situations.
Manufacturing companies can also benefit from data lakes, which can turn a flow of unstructured data into valuable insights and analytics on supply chains, electricity costs, and more.
Data lakes in the government and public sector can store unstructured data, such as census data and public records, to help policymakers make informed decisions about laws and regulations.
5 Signs Your Home May Be a Swamp
Your home may be a swamp if it's filled with clutter and disorganization. This can make it difficult to find things you need, much like a data lake with poor data quality.
A lack of clear labels and categorization can also turn your home into a swamp. This is similar to a data lake with poor metadata, making it hard for users to understand the data.
If you're constantly struggling to find what you need in your home, it may be a sign that you need to implement better systems for organization and management. This is similar to the need for regular audits and implementing best practices to maintain data integrity in a data lake.
Your home can become a swamp if it's filled with unnecessary items that take up space and make it hard to navigate. This is similar to the difficulties in data retrieval mentioned earlier.
If you're feeling overwhelmed and frustrated with the state of your home, it may be a sign that you need to take a step back and reassess your organization and management systems. This is similar to the need for better management and governance in a data lake.
Use Cases by Industry
Data lakes are versatile and can be applied to various industries, making them a valuable tool for organizations across different sectors.
In healthcare, data lakes can store unstructured data such as patient records, medical images, and genomic data. This allows healthcare organizations to analyze large amounts of data and gain insights into patient outcomes, disease patterns, and treatment effectiveness.
Government and public sector organizations can use data lakes to store unstructured data such as census data, public records, and data on public services like electrical grids. This enables policymakers and experts to make informed decisions about laws, regulations, and standards.
Manufacturing companies can utilize data lakes to analyze unstructured data from various sources, such as supply chains, electricity costs, and transportation. This helps manufacturers make data-driven decisions and gain insights into their business operations.
Banking and capital markets can use data lakes to store unstructured data from various sources, such as algorithmic trading, customer engagement, and business planning. This enables traders to make informed decisions and gain insights into market trends.
Here's a summary of data lake use cases by industry:
AI/ML Projects: Pilot to Production
AI/ML projects can be a game-changer, but scaling them across a business is a major challenge. 84% of business executives believe they need to use AI to achieve their growth objectives.
Deploying machine learning models automatically and governing them in a data lake is crucial. Companies that can't do this can't gain measurable value from AI.
No-code/low-code machine learning operations (MLOps) help data engineers operationalize ML models. This eliminates ad-hoc scripting and manual processes.
Only 24% of companies have successfully scaled AI across their business. This is a significant gap that needs to be addressed.
Companies need to find a way to automate the deployment of ML models. Otherwise, they'll struggle to achieve their growth objectives.
Frequently Asked Questions
Who is the owner of Data Lake, Inc.?
Data Lake Inc. was founded by Henry Sy Jr. and Anthony Almeda. They are the co-founders behind the company's vision for better connectivity and data management.
Sources
- https://www.alation.com/blog/data-swamp-lake-lakehouse/
- https://azure.microsoft.com/en-us/solutions/data-lake
- https://www.informatica.com/resources/articles/how-data-lakes-can-help-your-business.html
- https://www.stitchdata.com/resources/what-is-data-lake/
- https://www.imperva.com/learn/data-security/data-lakes/
Featured Images: pexels.com