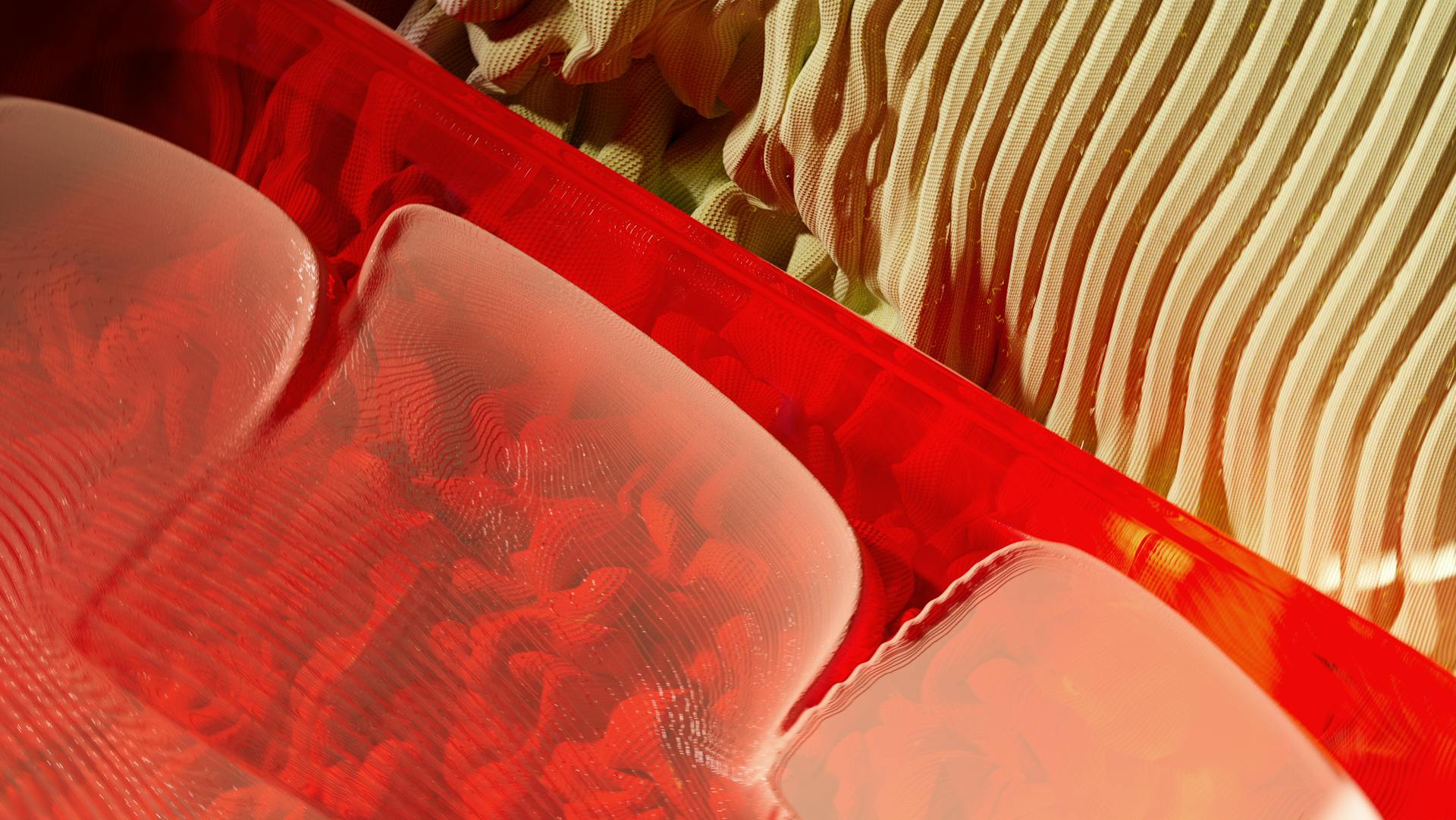
Accurate data collection is crucial for making informed decisions. Poor data quality can lead to misinformed decisions, which can have serious consequences.
Inaccurate data can be costly, with a study citing that inaccurate data can cost a company up to 20% of its revenue. This highlights the importance of accurate data collection.
To achieve accurate data collection, it's essential to have a clear understanding of what data is needed and how it will be used. This involves defining the scope and objectives of the data collection process.
Additional reading: Data Collection Endpoint Azure
Benefits of Accurate Data Collection
Accurate data collection is crucial for businesses to make informed decisions. It enables better AI implementation, as algorithms are only as good as the data used to train them.
Having accurate data makes it easier to identify core problems, as errors are often caused by other errors, making it difficult to trace where the root issue occurred. This leads to more reliable outputs and faster problem-solving.
Accurate data also provides a competitive advantage, as businesses can spot and take advantage of opportunities faster than their rivals. With quality data, your organization may be able to stay ahead of industry trends.
Improved customer service is another benefit of accurate data collection. By understanding customer preferences and interests, businesses can prepare for their needs and build a loyal customer base.
Accurate data collection also increases ROI, as it reduces the costs associated with identifying and fixing bad data. This frees up resources to focus on more productive tasks, like helping business users and data analysts take advantage of the available data.
Here are some specific benefits of accurate data collection:
- Better AI implementation
- Easier identification of core problems
- Competitive advantage
- Improved customer service
- Increased ROI
These benefits ultimately lead to better decision-making, operational efficiency, and improved internal processes. By prioritizing accurate data collection, businesses can streamline operations, boost sales, and deliver a competitive edge.
Challenges in Data Collection
Collecting accurate data is crucial, but it's not always easy. Human error is a common cause of inaccuracies in data, even with the most detail-oriented and careful people.
Manual data entry is a significant contributor to these errors, as it's prone to mistakes, especially when dealing with large amounts of data. No system is completely immune to human error, so it's essential to install data validation rules and regularly test them to ensure they're working properly.
To mitigate this risk, organizations should put controls in place to manage who can access and edit certain data, reducing the risk of unauthorized tampering.
Here are some common causes of manual data entry errors:
- Human error in transcribing data
- Increasing data volume and complexity
- Multiple people accessing and editing data
A lack of data standardization also contributes to poor data quality. Without clear guidelines on how to classify and format different types of data, organizations risk inconsistencies and errors. This can lead to sorting and analysis problems, making it difficult to trust the accuracy of the data.
Manual Entry
Manual Entry can be a recipe for disaster, especially when it comes to data accuracy. Human error is a common cause of inaccuracies in data.
No matter how detail-oriented and careful someone is, they are still at risk of making mistakes when transcribing data. This risk increases with the more data a person has to manage, as well as with the number of people who are allowed to access and edit data.
You can install systems in your organization’s databases to check for common input errors, such as spell checking and validation rules. These systems can help reduce the risk of data inaccuracies.
It’s also a good idea to put controls in place to manage who can access and edit certain data in your organization. This reduces the risk of someone who shouldn’t be editing your company’s data tampering with it.
Related reading: Why Is Being Organized Important
Lack of Standardization
Data quality issues can arise from a lack of standardization, leading to problems with sorting and analysis.
A lack of validation standards can cause data to be correct but still cause issues. This is due to formatting changes between similar records or multiple versions of the same record.
Uppercase vs. lowercase letters, punctuation, abbreviations, units of measurement, and date formats can all contribute to data quality problems.
Examples of date formats that can cause issues include 4/3/2022 being interpreted as either April 3rd or March 4th.
Clear guidelines on how to classify different types of data and what format each one should be in can help prevent data quality issues.
Recommended read: Why Is It Important to Keep Software up to Date
Decay
Data decay is a real issue that can undermine the value of your data. Data decay occurs when the status of something in the real world changes, making data that refers to it no longer accurate or relevant.
This usually happens when certain data is not used or accessed for an extended period of time. Investing too heavily in data collection can lead to data decay.
Having a diligent data team that stays on top of potential changes to data and revises it regularly can help address this problem. Investing in automated data management systems and/or dedicated data quality tools can also be beneficial.
Focusing on collecting relevant and accurate data for your business is a more general way to address data decay.
Recommended read: Is Billing Address Important
Siloing
Siloing is a major challenge in data collection. Data siloing occurs when data someone needs is inaccessible due to lack of authorization or knowledge of its existence within the organization.
This can lead to employees searching for comparable data from outside sources, causing data consistency issues. Duplicate records can arise, especially if the outside data differs in content or format from what the organization already has on file.
A well-defined system of validation rules and categorization can help reduce inconsistencies. This ensures that data is standardized and easily accessible.
Investing in a dedicated data catalog solution can also be beneficial. It helps employees know what data is available, evaluate its relevance, and gain seamless access to it.
For your interest: Why Is Learning and Development Important for Employees
Poor Culture
A poor culture can lead to data inaccuracy, and it's often caused by employees not understanding the importance of data quality. Traditionally, data quality has been seen as the responsibility of IT teams and BI specialists.
Employees who aren't trained to pay attention to data quality can inadvertently cause errors, which may only be addressed after a costly mistake has occurred. This is because they focus on their tasks without realizing the impact of their actions on data accuracy.
It's crucial that all members of a business, not just IT and BI teams, are educated on why data quality is important. This education should include how to maintain data accuracy in their daily work, as well as how to use modern data quality tools to clean and manage data.
As data becomes increasingly essential to modern business decisions, it's more important than ever to have a culture that values data quality.
Discover more: The Most Important Aspect S of a Company's Business Strategy
5 Factors That Affect
Data quality is a multifaceted concept that encompasses more than just accuracy. In fact, there are five key factors that affect how reliable data is for various use cases.
Accuracy is just one piece of the puzzle, and it's often difficult to judge the quality of data that isn't available in the first place. Completeness is a major concern, as missing data can make it harder to draw reliable conclusions.
If this caught your attention, see: Why Is Accuracy Important
Relevance is also crucial, as quality data can still be unhelpful if it doesn't answer the question(s) your organization is interested in. Having clear intentions on what you want to learn and why helps you focus on the right data.
Validity is another important aspect of data quality, ensuring that you can reasonably compare similar types of data. This includes presenting data in consistent formats and using the same units of measurement.
Timeliness is closely tied to data accuracy, as the shorter the time period between data production and collection, the more likely the data is to remain accurate. Conversely, the longer it's been since the event data refers to has occurred, the more likely conditions have changed and the data is no longer relevant.
Consistency is related to precision, referring to how often data is accurate across multiple datasets. This can make it difficult for departments within the same company, or multiple cooperating companies, to work together efficiently.
Here are the five key factors that affect data quality in a concise format:
- Completeness: Having all necessary data to draw reliable conclusions
- Relevance: Answering the question(s) your organization is interested in
- Validity: Presenting data in consistent formats and using the same units of measurement
- Timeliness: Collecting data close to when it's produced
- Consistency: Accurate data across multiple datasets
Emerging Challenges
Data quality efforts have become increasingly complex due to the rise of cloud computing and big data initiatives. Many organizations now work with both on-premises and cloud systems, making it harder to manage data quality.
A growing number of organizations are incorporating machine learning and other AI technologies into their operations and products, adding to the complexity of managing data. This is because these algorithms are often only as good as the data used to train them.
Data scientists are implementing complex data pipelines to support their research and advanced analytics, which can lead to data quality issues if not managed properly. Real-time data streaming platforms that continuously funnel large volumes of data into corporate systems also pose a challenge.
Data privacy and protection laws such as the European Union's General Data Protection Regulation (GDPR) and the California Consumer Privacy Act (CCPA) are giving people the right to access the personal data that companies collect about them. This means organizations must be able to find all of the records on an individual in their systems without missing any because of inaccurate or inconsistent data.
Consider reading: Why Is Data Protection Important
Here are some additional challenges that organizations face when it comes to data quality:
- Many organizations are working with both on-premises and cloud systems.
- Machine learning and other AI technologies are being incorporated into operations and products.
- Data scientists are implementing complex data pipelines.
- Real-time data streaming platforms are continuously funneling large volumes of data into corporate systems.
These challenges highlight the need for organizations to prioritize data quality and implement effective data management strategies to ensure the accuracy and consistency of their data.
Ensuring Data Accuracy
Ensuring data accuracy is crucial for making informed decisions and building trust with customers and stakeholders. Consistent data entry protocols, such as standardizing data entry procedures and training employees, can significantly reduce errors.
Automating data validation and cleansing processes is another effective way to minimize errors. Regular audits, including internal checkpoints and reviews, can also help flag and correct inaccuracies.
To foster a culture of accuracy, establishing a data governance framework is essential. This involves creating policies and procedures for data entry, validation, and maintenance to ensure ongoing accuracy. By following these best practices, your business can operate more efficiently and make smarter decisions.
Here are some key best practices for ensuring data accuracy:
- Consistent data entry protocols
- Automation tools
- Regular audits
- Data governance framework
By adopting these best practices, you can ensure your data remains accurate and reliable, laying the groundwork for future success.
Ensure
To ensure data accuracy, it's essential to adopt best practices that foster a culture of accuracy within your organization. Consistent data entry protocols, such as standardizing data entry procedures and training employees to minimize errors, are crucial.
Automation tools can significantly reduce errors by automating data validation and cleansing processes. Regular audits, including enforcing internal checkpoints and reviews, are also vital to flag and correct inaccuracies.
A data governance framework is necessary to establish policies and procedures for data entry, validation, and maintenance. This framework ensures ongoing accuracy and helps your business operate more efficiently, make smarter decisions, and build trust with customers and stakeholders.
To streamline your data processes, consider using Similarweb's Data-as-a-Service solutions, which provide accurate and real-time data insights.
Here are the key steps to ensure data accuracy:
- Standardize data entry procedures
- Automate data validation and cleansing processes
- Regularly audit your data
- Establish a data governance framework
By following these best practices, you can ensure your data remains accurate and reliable, laying the groundwork for future success.
Uniqueness
Uniqueness is a data quality characteristic most often associated with customer profiles. A single record can be all that separates your company from winning an e-commerce sale and beating the competition.
Greater accuracy in compiling unique customer information is often the cornerstone of long-term profitability and success.
Accurate customer information can help you tailor your marketing campaigns to individual customers, increasing the likelihood of a sale.
This is especially true for companies that rely on customer performance analytics related to individual company products and marketing campaigns.
By focusing on uniqueness, you can make data-driven decisions that drive business growth and success.
Check this out: Why Is Customer Success Important
Data Collection Best Practices
Accurate data collection is the backbone of any successful business. Consistent data entry protocols are essential to minimize errors, and automation tools can significantly reduce errors by automating data validation and cleansing processes.
To ensure data accuracy, regular audits should be conducted to flag and correct inaccuracies. This includes enforcing internal checkpoints and reviews. A data governance framework should also be established to ensure ongoing accuracy.
Adopting these best practices fosters a culture of accuracy within your organization, significantly reducing the chances of data errors. Your business can operate more efficiently, make smarter decisions, and build trust with customers and stakeholders.
To streamline your data processes, consider the following best practices:
- Establish employee and interdepartmental buy-in across the enterprise.
- Set clearly defined metrics.
- Ensure high data quality with data governance by establishing guidelines that oversee every aspect of data management.
- Create a process where employees can report any suspected failures regarding data entry or access.
- Establish a step-by-step process for investigating negative reports.
- Launch a data auditing process.
- Establish and invest in a high-quality employee training program.
- Establish, maintain, and consistently update data security standards.
- Assign a data steward at each level throughout your company.
- Leverage potential cloud data automation opportunities.
- Integrate and automate data streams wherever possible.
Data Management and Governance
Accurate data collection is crucial for any organization, and it all starts with data management and governance. Data governance is a data management system that adheres to internal standards and policies for collecting, storing, and sharing information. This ensures that all data is consistent, trustworthy, and free from misuse.
Data quality management tools can help streamline efforts by matching records, deleting duplicates, and validating new data. These tools can also perform data profiling, which examines, analyzes, and summarizes data sets. Many tools now include augmented data quality functions that automate tasks and procedures using machine learning and AI technologies.
To ensure data quality, organizations should establish employee and interdepartmental buy-in across the enterprise. This means setting clearly defined metrics and ensuring high data quality with data governance by establishing guidelines that oversee every aspect of data management. A data steward at each level throughout the company can also help oversee specific data sets.
Worth a look: Important Sets
The Plan-Do-Check-Act (PDCA) cycle is a framework that can be used to implement data governance. This cycle involves planning, executing, checking, and acting on data governance initiatives. Data governance practices can also be enhanced by establishing a data auditing process and launching a data quality management system.
Here are some best practices for ensuring data accuracy:
- Consistent data entry protocols: Standardize data entry procedures and train employees to minimize errors.
- Automation tools: Automating data validation and cleansing processes can significantly reduce errors.
- Regular audits: Regularly audit your data to flag and correct inaccuracies.
- Data governance framework: Establish policies and procedures for data entry, validation, and maintenance to ensure ongoing accuracy.
By following these best practices, organizations can foster a culture of accuracy and significantly reduce the chances of data errors. This, in turn, enables businesses to operate more efficiently, make smarter decisions, and build trust with customers and stakeholders.
Data Quality and Integrity
Data accuracy is the foundation of reliable data management, but it's not the only thing that matters. Data accuracy refers to the correctness of data when it's collected.
Data integrity, on the other hand, focuses on maintaining that quality over time. It ensures the data is reliable and trustworthy, true and factual.
Data quality and data integrity are closely related concepts in data management. Data quality ensures the overall accuracy, completeness, consistency, and timeliness of data, making it fit for its intended use.
Check this out: Is Memory Integrity Important
Vs. Integrity
Data accuracy and data integrity are not interchangeable terms, but rather two crucial aspects of reliable data management. Data accuracy refers to the correctness of data when it's collected.
Data accuracy is the foundation for data integrity, which focuses on maintaining data quality over time. Consider accuracy the starting point for a robust data management system.
Data quality and data integrity are sometimes used interchangeably, but they have distinct meanings. Data quality is focused on the data's ability to serve its specified purpose.
Data integrity, on the other hand, encompasses both logical and physical validity. Logical integrity includes data quality measures and referential integrity, which ensures related data elements are valid.
Physical integrity is concerned with access controls and security measures to prevent data modification or corruption by unauthorized users. It also involves protections like backups and disaster recovery.
In a broader sense, data integrity combines data quality, data governance, and data protection into a unified effort for addressing data accuracy, consistency, and security.
Consider reading: Why Is Data Integrity Important
Validity
Validity is about following specific company formats, rules, or processes. For example, if a customer doesn't enter their birthdate using the proper format, data quality becomes compromised.
Data validity is often tested by systems that reject information unless it meets pre-assigned requirements. Many organizations design their systems to reject data that doesn't fit the format.
Validity is an important aspect of data quality, and it's essential to have clear rules and processes in place to ensure data accuracy. Without these rules, data quality suffers and can lead to errors and inconsistencies.
Systems can be designed to automatically reject data that doesn't meet specific requirements, making it easier to maintain data quality. This can save time and resources in the long run.
Data validity is closely related to data integrity, which ensures that data is reliable and trustworthy. By prioritizing data validity, organizations can improve their overall data quality and integrity.
A fresh viewpoint: Why Is Integrity Important in Your Professional Life
Sources
- https://www.similarweb.com/blog/daas/data-analysis/data-accuracy/
- https://www.safegraph.com/guides/why-accurate-data-is-important
- https://www.techtarget.com/searchdatamanagement/definition/data-quality
- https://www.alation.com/blog/what-is-data-quality-why-is-it-important/
- https://www.forbes.com/sites/forbesinsights/2017/06/05/the-importance-of-data-quality-good-bad-or-ugly/
Featured Images: pexels.com