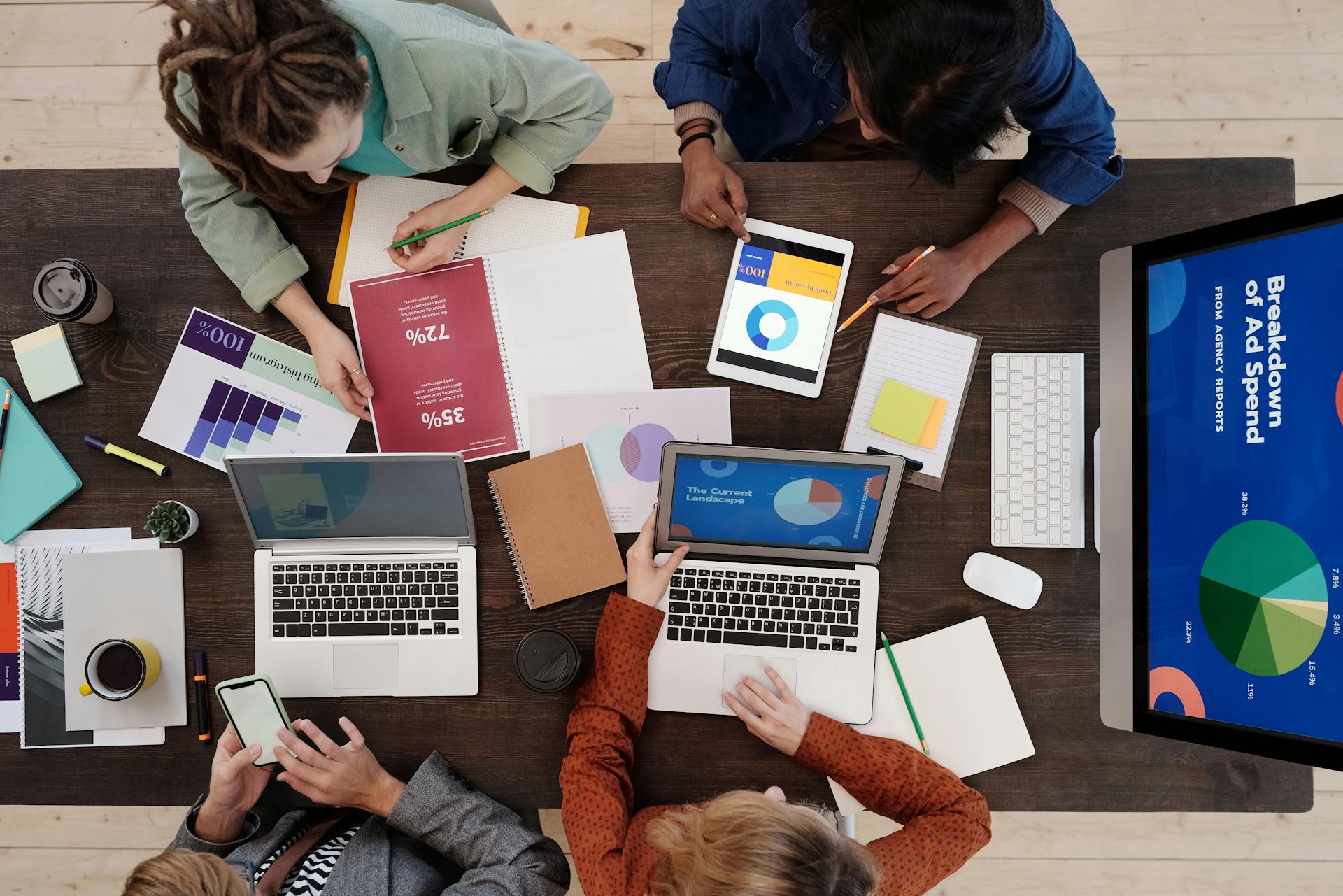
Statistical significance is a crucial concept in data analysis that can make or break the conclusions we draw from our findings.
A low p-value, typically below 0.05, indicates that the results are statistically significant, meaning they are unlikely to occur by chance.
Statistical significance matters because it helps us distinguish between real effects and random fluctuations in the data.
In a study with a large sample size, a small effect can still be statistically significant if the sample size is large enough.
A statistically significant result doesn't necessarily mean the effect is practically significant, meaning it may not be large enough to be meaningful in real-world terms.
For instance, if a new medicine is found to be statistically significant in reducing blood pressure, but the reduction is only 1 mmHg, it may not be practically significant enough to make a noticeable difference in patients' lives.
Take a look at this: Significant Important
What Is
Statistical significance is a determination made by an analyst that the results in data aren't explainable by chance alone. This is often used in fields like medicine, physics, and biology to determine the effectiveness of a new treatment or product.
Statistical significance can be considered strong or weak, with a small p-value indicating strong statistical significance. A p-value of 5% or lower is often considered to be statistically significant.
Statistical significance is a measure of how unusual your experiment results would be if there were actually no difference in performance between your variation and baseline. Achieving statistical significance helps ensure that conclusions drawn from experiments are reliable and not based on random fluctuations in data.
Most experiments fail to reach a substantial significance level due to changes being too small, low baseline conversion rates, or too many goals being tracked. These issues can lead to test results showing significant standard deviations, making it difficult to draw reliable conclusions.
A p-value is the probability of observing results as extreme as those in the data assuming the results are truly due to chance alone. If a p-value is small, then the result is considered more reliable.
Here are some common reasons why experiments may fail to reach statistical significance:
- Changes are too small: Most changes to visitor experience aren’t impactful and they fail to reach clinical significance due to sampling error.
- Low baseline conversion rates: Most data sets use metrics with low baseline as a proxy which often results in test results showing significant standard deviations.
- Too many goals: Often, teams don’t focus on crucial metrics aligned with their hypothesis, resulting in research findings falling short of the significance threshold.
Importance of Statistical Significance
Statistical significance is a crucial concept in understanding the results of experiments and data analysis. It determines whether the results are due to chance alone or if there's a more systematic explanation.
Statistical significance is often used in pharmaceutical drug trials, vaccine testing, and pathology research to test for effectiveness. It's a way to inform investors about a company's success in releasing new products.
A p-value is a key factor in determining statistical significance. A small p-value, typically 5% or less, indicates that the results aren't easily explainable by chance alone.
In classical statistics, a strict set of guidelines must be followed to get valid results from experiments. These include setting a minimum detectable effect and sample size in advance, not peeking at results, and not testing too many goals or variations at the same time.
Stats Engine, an advanced statistical model, can help determine practical significance by calculating the estimated duration of an experiment. This involves multiplying the sample size by the number of variations and then dividing by the average daily visitors.
Check this out: The Most Important Aspect S of a Company's Business Strategy
Here's a simple formula to calculate the estimated duration of an experiment:
- Total visitors needed = Sample size × Number of variations
- Estimated days to run = Total visitors needed ÷ Average daily visitors
With Stats Engine, statistical significance should generally increase over time as more evidence is collected. This evidence comes in the form of larger conversion rate differences and conversion rate differences that persist over more visitors.
Intriguing read: Is Refresh Rate Important for Tv
Calculating and Interpreting Statistical Significance
Calculating statistical significance is a crucial step in determining whether the results of an experiment or study are due to chance or something more. Statistical significance is calculated using the cumulative distribution function, which tells you the probability of certain outcomes assuming that the null hypothesis is true.
The null hypothesis is the hypothesis that no effect exists in the phenomenon being studied. To determine whether the null hypothesis should be rejected, a researcher calculates a p-value, which is the probability of observing an effect of the same magnitude or more extreme given that the null hypothesis is true. The null hypothesis is rejected if the p-value is less than (or equal to) a predetermined level, α, which is usually set at or below 5%.
For your interest: Why Is Customer Lifetime Value Important
Statistical significance is determined by comparing the p-value to the predetermined significance level, α. If the p-value is sufficiently small, typically 5% or less, the data is deemed inconsistent with the null hypothesis and the null hypothesis is rejected. This means that the results are not easily explained by chance alone and a more systematic explanation is needed.
How to Calculate It
Calculating statistical significance involves determining the probability of certain outcomes assuming the null hypothesis is true. This is done using the cumulative distribution function. If researchers determine that this probability is very low, they can eliminate the null hypothesis.
To calculate statistical significance, you need to determine the p-value, which is the probability that an observed difference could have occurred just by random chance. A p-value is a measure of the probability that an observed difference could have occurred just by random chance. The results are not easily explained by chance alone and the null hypothesis can be rejected when the p-value is sufficiently small, 5% or less.
Related reading: What Is an Important Difference between Statistics and Parameters
Statistical significance is often determined using statistical hypothesis testing, where researchers attempt to support their theories by rejecting other explanations. Statistical hypothesis testing is used to determine whether data is statistically significant and whether a phenomenon can be explained as a byproduct of chance alone.
To determine whether a result is statistically significant, a researcher calculates a p-value, which is the probability of observing an effect of the same magnitude or more extreme given that the null hypothesis is true. The null hypothesis is rejected if the p-value is less than (or equal to) a predetermined level, α, which is also called the significance level, and is the probability of rejecting the null hypothesis given that it is true (a type I error). It is usually set at or below 5%.
Here's a quick rundown of the steps involved in calculating statistical significance:
- Determine the null hypothesis
- Calculate the p-value
- Compare the p-value to the predetermined significance level (α)
- Reject the null hypothesis if the p-value is less than (or equal to) α
By following these steps, you can determine whether your results are statistically significant and make informed decisions based on your data.
Effect Size
Effect size is a measure of a study's practical significance. It's a way to gauge the research significance of a result, and it's especially useful when a statistically significant result has a weak effect.
A statistically significant result may have a weak effect, which is why researchers are encouraged to report an effect size along with p-values. This helps to provide a complete picture of the study's findings.
An effect size measure quantifies the strength of an effect, such as the distance between two means in units of standard deviation. For example, Cohen's d is a measure of effect size that compares the distance between two means in units of standard deviation.
The correlation coefficient between two variables is another measure of effect size. Its square can also be used to indicate the strength of the relationship between the variables.
Effect size is an important consideration in research, as it helps to determine the practical significance of a study's findings.
For your interest: Why Is It Important to Study Communication
Understanding and Applying Statistical Significance
Statistical significance is a crucial concept in research, but it's often misunderstood. Statistical significance is a determination of the null hypothesis, which suggests that the results are due to chance alone. A data set provides statistical significance when the p-value is sufficiently small.
The p-value indicates the probability under which the given statistical result occurred, assuming chance alone is responsible for the result. If this probability is small, then the researcher can conclude that some other factor could be responsible for the observed data. The p-value is a function of the means and standard deviations of the data samples.
A p-value of 5% or less is typically considered statistically significant. The opposite of the significance level, calculated as 1 minus the significance level, is the confidence level. It indicates the degree of confidence that the statistical result did not occur by chance or by sampling error.
To determine statistical significance, researchers use a strict set of guidelines, including setting a minimum detectable effect and sample size in advance, not peeking at results, and not testing too many goals or variations at the same time. These guidelines can be cumbersome, but they help produce valid results.
Broaden your view: Why Digital Marketing Is Important for Small Business
Here are the different types of statistical significance tests:
- One-tailed test: Used when the research question or alternative hypothesis specifies a direction.
- Two-tailed test: Used when the research question or alternative hypothesis does not specify a direction.
- Parametric tests: Used for normally distributed data.
- Non-parametric tests: Used for non-normally distributed data.
A high level of statistical significance does not prove that a hypothesis is true or false. It only measures the likelihood that an observed outcome would have occurred, assuming that the null hypothesis is true.
Best Practices and Limitations
Statistical significance is crucial in research because it helps identify patterns and trends that are likely to be real.
A common mistake is to rely too heavily on sample size, which can lead to false positives.
The p-value is a measure of statistical significance, and a common threshold is 0.05, meaning there's only a 5% chance of obtaining the results by chance.
To determine the sample size needed for a study, researchers can use statistical power, which is the probability of detecting an effect if it exists.
Statistical significance does not guarantee practical significance, meaning a significant result may not be meaningful in real-world terms.
Thresholds in Specific Fields
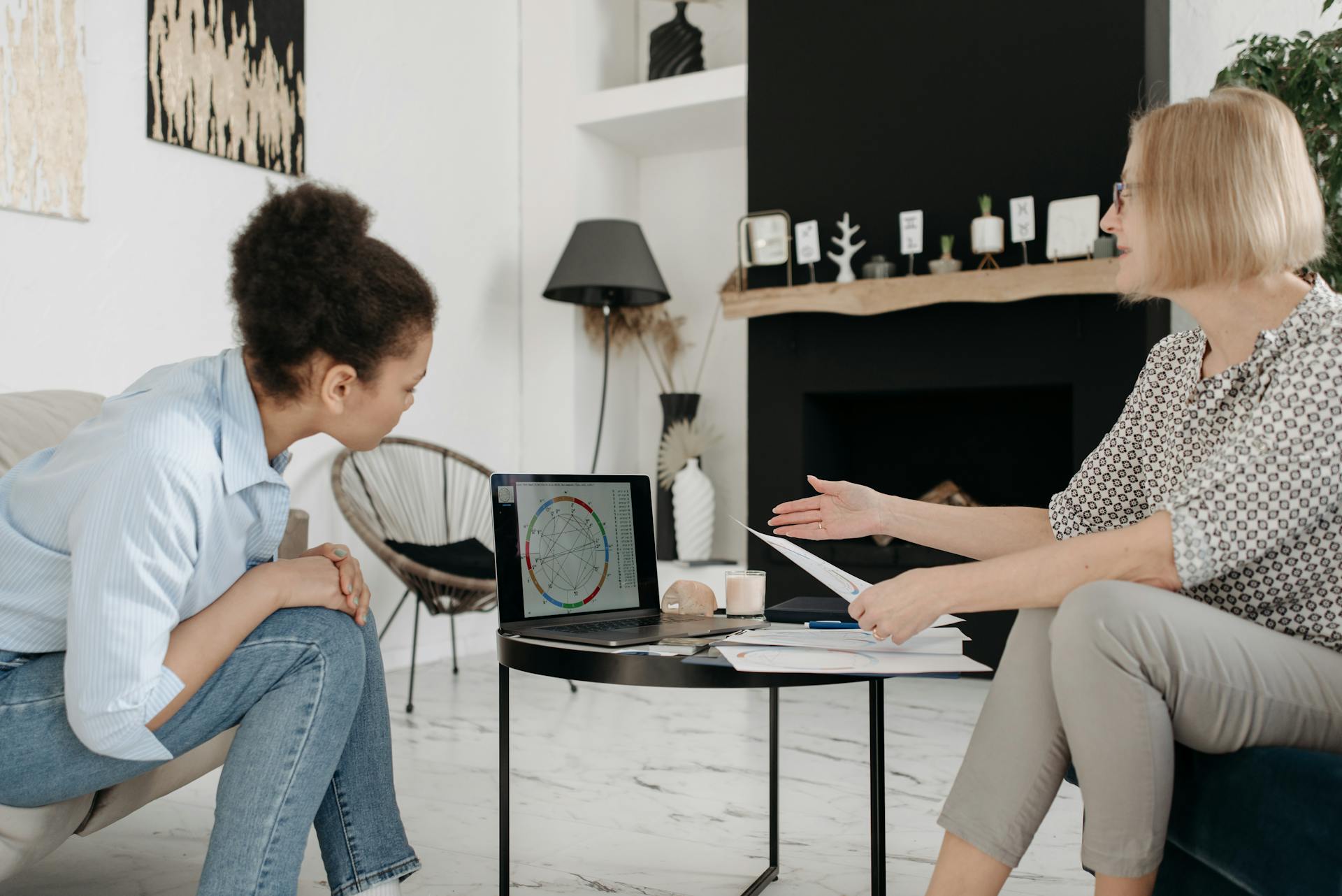
In particle physics and manufacturing, statistical significance is often expressed in multiples of the standard deviation or sigma (σ) of a normal distribution.
Significance thresholds in these fields are set at a much stricter level, typically 5σ, which corresponds to a p-value of about 1 in 3.5 million.
In genome-wide association studies, significance levels as low as 5×10 are not uncommon.
The extremely large number of tests performed in these studies means that even low significance levels can be statistically significant.
Best Practices for Reaching
Reaching statistical significance can be a challenge, but there are some best practices you can follow to increase your chances of success. One key practice is to run tests for at least one business cycle, which is typically 7 days.
This allows you to collect enough data to get a reliable reading. I've seen many tests fail to reach significance because they were run for too short a period.
Broaden your view: One Important Purpose of a Brand Is to
To choose the right metrics, select primary and secondary metrics carefully. This will help you focus on the most important factors and avoid unnecessary complexity.
Here are some key metrics to consider:
- Primary metrics: These are the main goals you're trying to achieve, such as conversion rates or revenue growth.
- Secondary metrics: These are secondary goals that support your primary metrics, such as user engagement or satisfaction.
Designing experiments with significant potential impact on user behavior is also crucial. This means thinking about the changes you're making and how they might affect users. By following these best practices, you can increase your chances of reaching statistical significance and getting valuable insights from your tests.
Limitations
Researchers focusing solely on statistical significance might report findings that are not substantive and not replicable. This can lead to studies that are statistically significant but lack practical significance, meaning they may not have a real-world impact.
A study can be statistically significant without being practically significant. This is a key distinction to keep in mind when evaluating research findings.
Statistical significance is not the same as practical significance. A study that is statistically significant may not necessarily be practically significant, meaning it may not have a meaningful or noticeable effect in the real world.
This difference can be crucial in determining the usefulness and applicability of research findings.
Additional reading: World Map with Important Cities
Enhancing Healthcare Team Outcomes
Having a cohesive understanding of statistical significance is crucial for healthcare team members to draw accurate conclusions from research studies. Each team member, including nurses, physicians, and pharmacists, needs to understand statistical significance to make informed decisions.
If team members don't have a harmonious understanding of statistical significance, they may draw opposing conclusions from the same research. This can lead to confusion and potentially harm patient care.
Healthcare team members need to peruse copious literature and consider conclusions based on statistical significance.
Here's an interesting read: Why Is Healthcare Marketing Important
Frequently Asked Questions
Is statistical significance meaningful?
Statistical significance indicates that a result is likely real and not due to chance, giving you confidence in its authenticity. However, it's essential to understand what it means and how it's calculated to truly grasp its significance.
Sources
- https://www.ncbi.nlm.nih.gov/books/NBK459346/
- https://www.investopedia.com/terms/s/statistical-significance.asp
- https://www.investopedia.com/terms/s/statistically_significant.asp
- https://en.wikipedia.org/wiki/Statistical_significance
- https://www.optimizely.com/optimization-glossary/statistical-significance/
Featured Images: pexels.com