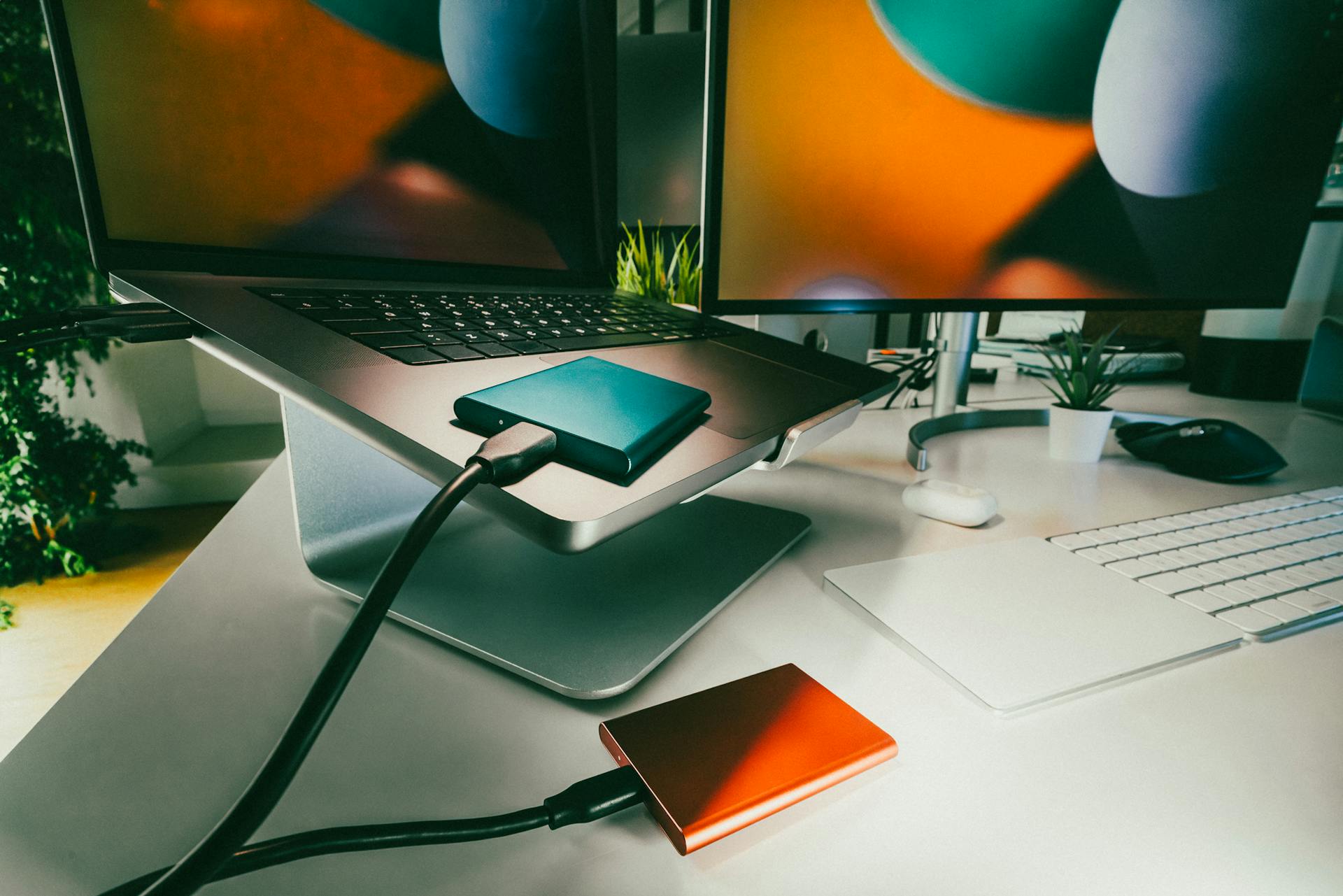
A marketing data lake is a centralized repository that stores all your marketing data in a single, easily accessible location. This allows you to have a 360-degree view of your customers and their interactions with your brand.
By consolidating your data into a single lake, you can eliminate data silos and reduce the time spent searching for and integrating data from multiple sources. This is especially important for marketing teams, who often rely on data to inform their decisions.
A marketing data lake can be thought of as a hub that connects all your marketing tools and systems, allowing you to analyze and derive insights from your data in real-time. This enables you to respond quickly to changes in the market and make data-driven decisions that drive business growth.
What Is a
A marketing data lake is a cloud-based storage system that aggregates both structured and unstructured marketing data from various sources in its raw form.
It acts as a reservoir of data droplets, each representing a piece of unprocessed marketing information, providing flexibility for future utilization. This is similar to a traditional database, but without strict table-row-column relationships.
A marketing data lake is a term for storing customer and business information, gathering data from multiple sources and storing, accessing, and analyzing information such as customer profiles, website metrics, and sales leads.
It's a centralized, single version of the truth for all customer touchpoints across your organization, making it a massive repository of customer data such as purchase history, demographics, and other information.
A marketing data lake is a storage system for storing, integrating, and analyzing all marketing data, essentially serving as a foundation for any analysis or experimentation that needs to happen on your company’s marketing channels.
It can capture, store, and manage all marketing campaign data, including ads and where they go after viewing them, allowing you to isolate critical trends and patterns, such as standard demographics, behaviors, or preferences.
Data Lake vs. Data Warehouse
A marketing data lake and a data warehouse are two distinct solutions for storing and analyzing data. A data warehouse is a structured repository optimized for data analysis and reporting, where data is cleaned, transformed, and loaded into the warehouse for querying and analysis.
The main difference between the two lies in their purpose. A data warehouse is designed to answer a specific business requirement or user need, whereas a data lake allows for flexible storage and analysis with a pending purpose.
Here's a comparison of the two:
This comparison highlights the key differences between a marketing data lake and a data warehouse. By understanding these differences, you can make informed decisions about which solution to implement based on your specific marketing goals and data requirements.
What Exactly Is?
A marketing data lake is a cloud-based storage system that aggregates both structured and unstructured marketing data from various sources in its raw form.
It acts as a reservoir of data droplets, each representing a piece of unprocessed marketing information, providing flexibility for future utilization.
A marketing data lake is similar to a traditional relational database like SQL Server or Oracle, except it doesn't require you to follow strict table-row-column relationships.
It's a platform that gathers data from multiple sources, storing, accessing, and analyzing information such as customer profiles, website metrics, and sales leads.
A marketing data lake is a centralized, single version of the truth for all customer touchpoints across your organization.
It's a massive repository of customer data such as purchase history, demographics, and other information.
The first step in working with a marketing data lake is to collect the data and select a sampling representing your entire audience.
Next is to isolate critical trends and patterns, such as standard demographics, behaviors, or preferences.
vs. a Warehouse
A data warehouse is optimized for data analysis and reporting, with data cleaned, transformed, and loaded (ETL process) into the warehouse before it's arranged in schemas suited for querying and analysis.
Check this out: Data Warehouse to Data Lake Migration
Data warehouses house structured, filtered, and processed data that is ready for analysis, with organization and indexing to facilitate easy search.
Data warehouses are designed from the ground up to solve a specific business requirement or user need, with little room for adaptability or analytical improvisation later.
The data in a data warehouse is primarily structured, with high query performance, optimized for querying, and preprocessed (ETL before storage).
Data warehouses can be pricier when scaling, but they offer high-quality data and compliance with data governance standards.
Here's a comparison of data lakes and data warehouses:
Data warehouses are ideal for fast, complex querying and analysis, but they can be less adaptable to changing business needs.
Benefits
Marketing data lakes offer a range of benefits that can transform the way marketers access and utilize data.
By storing any kind of data, resource savings can be achieved, with no loss of value. This is because resources are only expended if and when information is consumed.
Data lakes provide a way to break down rigid silos and bureaucratic boundaries between business processes, empowering every stakeholder to access any and all enterprise data if they have the proper privileges.
This flexibility ensures that marketers can capture the full spectrum of insights without being confined by data formats, whether it's structured data, semi-structured clickstream data, or unstructured social media content.
Data lakes can store diverse data types, making it easier to capture insights from various sources.
Storing vast amounts of data can be costly, but marketing data lakes offer a more cost-effective solution by distributing data across multiple servers, achieving better storage rates.
Here are the key benefits of marketing data lakes:
- Resource reduction: saving time and resources by storing data without unnecessary processing.
- Organization-wide accessibility: breaking down silos and bureaucratic boundaries to empower stakeholders.
- Performance efficiency: simpler data pipelines and faster design and planning processes.
- Cost-Efficiency: storing vast amounts of data without incurring prohibitive costs.
Potential Drawbacks and Challenges
Marketing data lakes can be a powerful tool for businesses, but they're not without their challenges. Data overload is a common issue, making it difficult to find specific information or trends in the vast amounts of data stored.
Here are some of the potential drawbacks and challenges of marketing data lakes:
- Data Overload: The sheer volume of data can make it challenging to find specific information or trends.
- Security Concerns: Ensuring data security against breaches requires constant vigilance and investment.
- Potential Disorganization: Lack of organization can scatter data, complicating the search for relevant information.
- Skill Requirements: Handling data lakes demands expertise, possibly necessitating specialized personnel.
- Integration Issues: Integrating data from diverse sources can present compatibility and synchronization challenges.
- Maintenance Costs: Maintaining data lakes involves regular updates and infrastructure management.
To avoid these challenges, it's essential to design and implement a data lake with careful planning and management. This includes setting business priorities, designating use cases and end users, committing to good communication, and establishing a robust data ingestion process. By taking these steps, businesses can ensure their data lake remains a valuable asset, rather than a data swamp.
Challenges
Data overload can make it challenging to find specific information or trends in a marketing data lake, as the sheer volume of data can be overwhelming.
Security concerns are a major issue, requiring constant vigilance and investment to ensure data security against breaches.
Potential disorganization can scatter data, complicating the search for relevant information in a data lake.
Handling data lakes demands expertise, possibly necessitating specialized personnel.
Integration issues can arise when integrating data from diverse sources, presenting compatibility and synchronization challenges.
See what others are reading: Security Data Lake
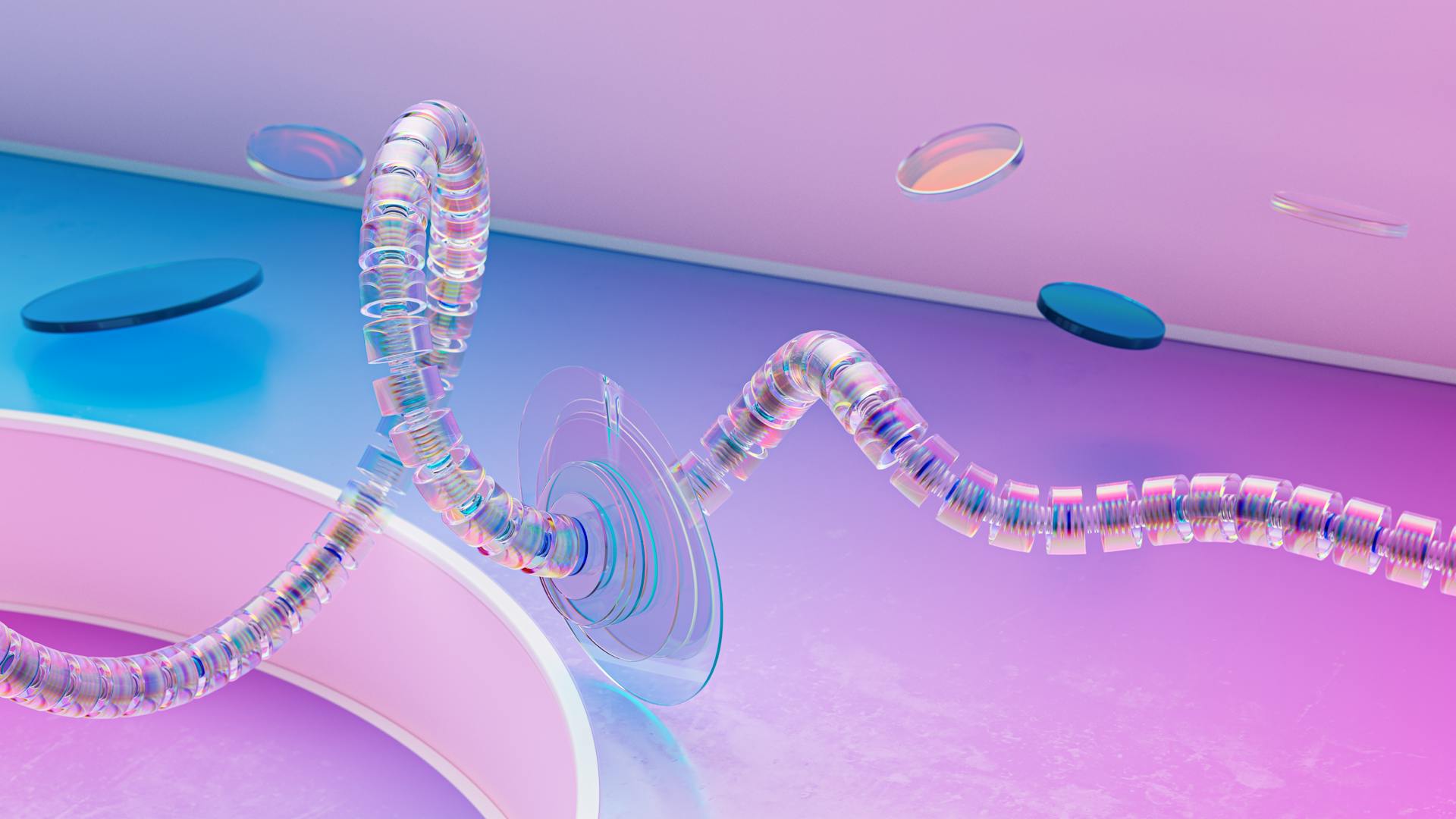
Maintenance costs can be high, involving regular updates and infrastructure management to keep the data lake running smoothly.
Here are some potential challenges to consider:
- Data Overload
- Security Concerns
- Potential Disorganization
- Skill Requirements
- Integration Issues
- Maintenance Costs
A data lake can become a data swamp if not properly managed, with degraded value due to design mistakes, stale data, or uninformed users and lack of regular access.
To avoid this, it's essential to set business priorities, designate use cases and end users, commit to good communication, and establish a robust data ingestion process.
A data lake cannot be opaque storage, and businesses must commit to good communication to maintain focus and ensure important stakeholders are aware of how and why to use the data in a data lake.
Establishing a robust data ingestion process is crucial, as getting uncorrupted raw data into storage is a primary focus.
Potential for increased costs can creep in as the volume and variety of data grow, leading to a need for more powerful processing capabilities.
Ensuring high availability and performance can lead to increased infrastructure and management expenses as the organization's reliance on the data lake increases.
For another approach, see: Create Azure Data Lake Storage Gen2
Security Concerns
Security Concerns are a major challenge when it comes to data lakes. Centralizing a company's data assets in a data lake can make it a prime target for security breaches.
Protecting sensitive data from unauthorized access is crucial. Companies must be proactive in implementing security measures to prevent data breaches.
Companies often need to make additional investments to ensure the security of their data lake. This can add layers of complexity to the data lake's management.
Ensuring compliance with data protection regulations is a significant concern for companies with data lakes. Data protection regulations can be complex and time-consuming to navigate.
Companies must take a proactive approach to security to protect their data lake from security breaches and unauthorized access.
Agility vs. Quality
Data lakes are ideal for agile analysis and machine learning because the raw data is malleable. In a data warehouse, the business processes used to assemble and manage the system ensure high-quality data.
The unstructured nature of data lakes means less strict adherence to data governance practices. This can be a challenge for organizations that require high-quality data.
Data lakes facilitate analytics, but their purpose might be pending, unlike data warehouses where processed data has a predetermined use case.
Check this out: Why Data Quality Is Important
Strategies for Success
To embark on a successful marketing data lake implementation, it's essential to define clear goals for your data lake's use. This will guide your data collection and analysis efforts, ensuring everyone is on the same page.
Choosing the right technology is also crucial. Select tools that match your needs and support diverse data types and real-time processing. This will enable you to handle various data formats and make data-driven decisions quickly.
A well-structured data governance plan is vital to maintaining data accuracy. Establish guidelines for accurate data entry, categorization, and regular audits to ensure your data lake remains reliable and trustworthy.
To safeguard your data, implement robust security measures to prevent breaches and unauthorized access. This will give you peace of mind and protect your business from potential data-related risks.
Here are the key strategies for a successful marketing data lake implementation:
- Defining Clear Goals
- Choosing Technology
- Data Governance
- Security Measures
- Team Collaboration
Strategies for Successful Implementation
To implement a successful marketing data lake, it's essential to define clear goals and use cases. This will guide your data collection and analysis efforts.
Setting specific objectives will help you determine what data to collect and how to analyze it. For example, are you aiming to enhance customer understanding, optimize campaigns, or refine targeting?
Choosing the right technology is also crucial. Select tools that match your needs and support diverse data types and real-time processing. This will ensure that your data lake can handle the complexity of marketing data.
Data governance is another critical aspect to consider. Establish guidelines for accurate data entry, categorization, and regular audits to prevent data quality issues. This will help maintain data integrity and ensure that your insights are based on trustworthy information.
Here are some key strategies for successful implementation:
- Define clear goals and use cases to guide data collection and analysis efforts.
- Choose technology that matches your needs and supports diverse data types and real-time processing.
- Establish data governance policies to ensure data quality and integrity.
- Implement security measures to safeguard data against breaches and unauthorized access.
- Foster collaboration and training among data analysts, marketers, and IT personnel for optimal insights.
By following these strategies, you can ensure a successful marketing data lake implementation and unlock the full potential of your marketing efforts.
Team Collaboration
Team collaboration is crucial for success. Regular training sessions keep everyone updated on the latest tools and methodologies.
Effective collaboration among team members, such as data analysts, marketers, and IT personnel, is key to deriving insights and driving data-driven decisions.
Foster a culture of collaboration where team members can share knowledge and expertise. This will lead to a more cohesive and productive team.
By keeping team members well-versed in the latest tools and methodologies, you'll be able to make informed decisions and stay ahead of the competition.
Frequently Asked Questions
What is the difference between a data lake and a CDP?
A Data Lake stores all types of data, while a Customer Data Platform (CDP) focuses on organizing and activating customer data for marketing and customer experience purposes. In essence, a Data Lake is a broader storage solution, whereas a CDP is a specialized platform for customer data.
What does a data lake do?
A data lake is a centralized repository that stores and processes large amounts of various data types in their native format, without size limits. It's a powerful tool for managing and analyzing complex data, learn more about how to leverage it for your business.
Sources
- https://improvado.io/blog/what-is-marketing-data-lake
- https://kiranvoleti.com/marketing-data-lake-with-insights
- https://www.marketdataforecast.com/market-reports/data-lake-market
- https://blog.bccresearch.com/the-5-leading-companies-in-the-data-lake-solutions-industry
- https://www.stitchdata.com/resources/what-is-data-lake/
Featured Images: pexels.com