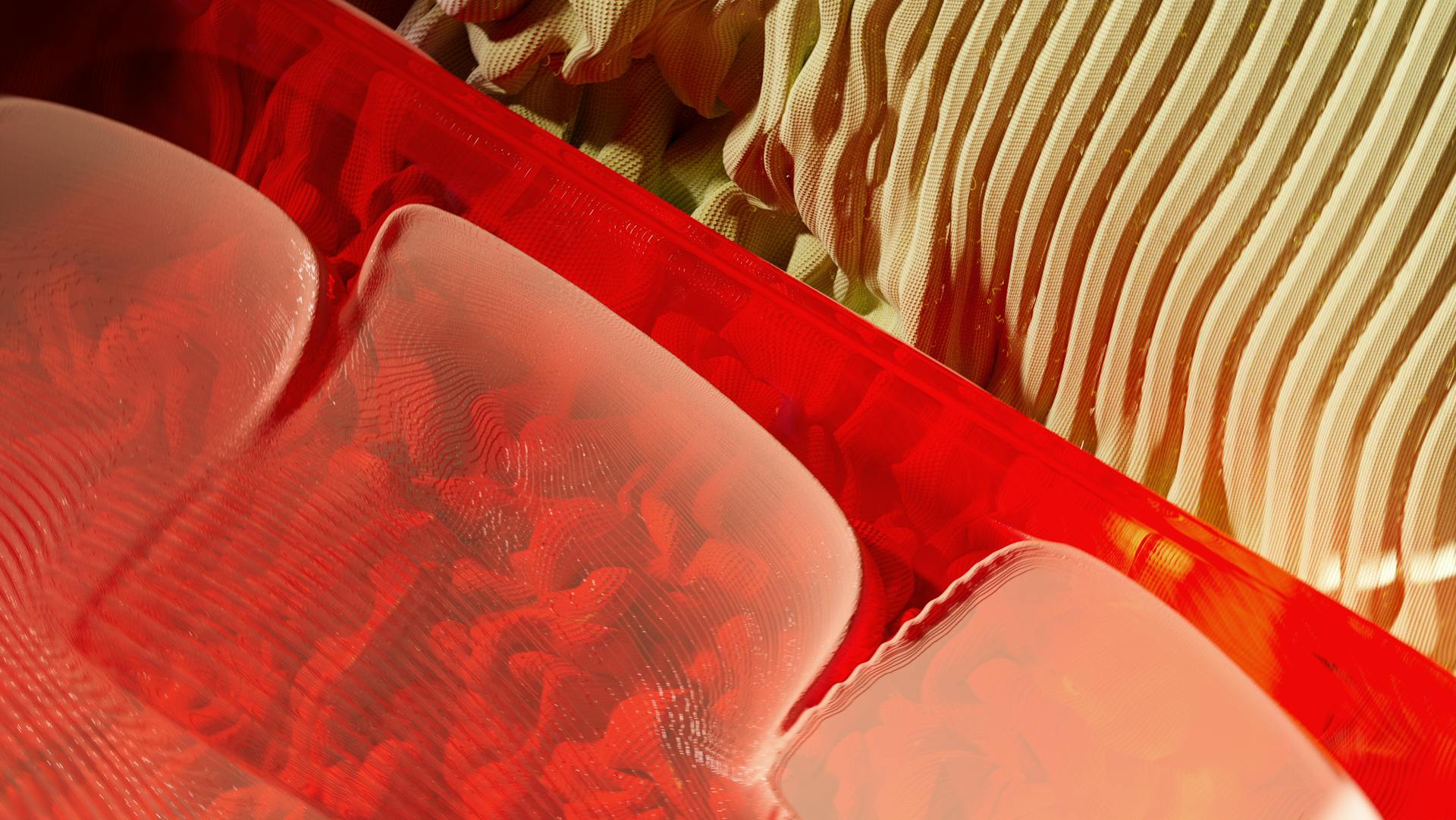
Ensuring data quality is crucial for business growth and efficiency. Poor data quality can lead to costly mistakes and lost revenue.
Inaccurate data can cause businesses to make decisions based on flawed assumptions, leading to financial losses and damaged reputations. For instance, a company that relies on outdated customer information may end up targeting the wrong audience with their marketing efforts.
Data quality issues can also hinder business efficiency by slowing down operations and increasing manual labor. This can be seen in the example of a company that spends a significant amount of time and resources correcting errors in their customer database.
Take a look at this: Why Are Values Important in a Company
Challenges in Data Quality
Data quality is a multifaceted challenge that affects organizations of all sizes. Emerging data quality challenges include managing structured, unstructured, and semi-structured data, as well as dealing with the complexity of on-premises and cloud systems.
Many organizations now work with both on-premises and cloud systems, which adds to the complexity of managing data. A growing number of organizations are also incorporating machine learning and other AI technologies into their operations and products, further complicating data quality efforts.
You might enjoy: Why Is a Target Market Important to Businesses and Organizations
Data quality concerns are also growing due to the implementation of data privacy and protection laws, such as the European Union's General Data Protection Regulation (GDPR) and the California Consumer Privacy Act (CCPA). These laws give people the right to access the personal data that companies collect about them, which means organizations must be able to find all of the records on an individual in their systems without missing any because of inaccurate or inconsistent data.
Here are some key factors that contribute to data quality challenges:
- Completeness: Missing data can make it difficult to draw reliable conclusions.
- Relevance: Data that doesn't answer the question(s) your organization is interested in is unhelpful.
- Validity: Data that is dissimilar or presented in different formats can be difficult to organize or analyze.
- Timeliness: Data that is collected and used too long after it was produced may no longer be accurate.
- Consistency: Inconsistent data across multiple datasets can make it difficult for departments or companies to work together efficiently.
5 Factors That Affect Data Quality
Data quality is a multifaceted concept that encompasses various factors that affect its reliability. Completeness is one such factor, and it refers to the percentage of data that is missing within a dataset. If a dataset is incomplete, it can be challenging to draw reliable conclusions from the data that is available.
Relevance is another crucial factor that affects data quality. Quality data can still be unhelpful if it doesn't answer the question(s) your organization is interested in. Before gathering data, it's essential to set clear intentions on what your organization wants to learn and why.
Validity is a critical aspect of data quality, ensuring that your organization can reasonably compare similar types of data. If data is dissimilar, including being presented in different formats or measured by different units, it can be difficult to organize or analyze properly.
Timeliness is closely tied to data accuracy and refers to the time between when data is produced and when it is collected and used. The shorter this time period, the more likely the data is to remain accurate.
Consistency is related to precision and refers to how often data is accurate across multiple datasets. Even if data is correct in one dataset, if it is different in content or format in another dataset, it can make it difficult for departments within the same company or multiple cooperating companies to work together efficiently.
Here are the five factors that affect data quality in a concise table:
Data quality is a critical aspect of any organization, and understanding these factors can help you improve the reliability of your data. By focusing on these factors, you can ensure that your data is accurate, complete, relevant, valid, timely, and consistent, ultimately leading to better decision-making and improved business outcomes.
See what others are reading: Important Factors
Consistency
Consistency is a crucial aspect of data quality. Data items should be consistent both in their content and format.
Different departments within a company may be operating under different assumptions about what is true, which can lead to poor coordination and even conflicting goals. This can be due to a lack of consistency in data collection and representation.
The Data Quality Assessment Framework (DQAF) organizes data quality dimensions into six major categories, including consistency. Most data managers assign a score of 0-100 for each dimension.
Inconsistent data can make it difficult to compare data items across multiple data sets or databases. This can lead to incorrect assumptions and poor decision-making.
For example, if a company has two similar pieces of information, such as the date on file for a customer's account and the last time they logged into their account, the difference in these dates can provide valuable insights into the success rates of marketing campaigns.
A different take: Why Is Lead Generation Important
Consistency ensures that the source of information collection is capturing the correct data based on the unique objectives of the department or company.
Here are some examples of inconsistent data formats that can cause problems:
- Uppercase vs. lowercase letters
- Punctuation
- Abbreviations
- Units of measurement
- Date formats (e.g. 4/3/2022 could be April 3rd or March 4th)
Siloing
Data siloing is a problem where data someone needs is locked away within an organization, inaccessible due to lack of authorization or even knowledge of its existence.
This can lead to employees seeking comparable data from outside sources, causing data consistency issues with duplicate records.
Data standardization can help reduce inconsistencies by having a well-defined system of validation rules and categorization for certain types of data.
Investing in a dedicated data catalog solution can help people in your organization know what data is available, evaluate its relevance, and access it seamlessly.
Data consistency issues arise when outside data differs in content or format from what an organization already has on file, making it a challenge to maintain accurate data.
Here's an interesting read: What Is an Important Factor That Help Determines Cost
Poor Culture
A poor culture within an organization can lead to data inaccuracy. This is because employees may not be trained to pay attention to data quality, leaving it to IT teams and BI specialists to handle.
Traditionally, data quality has been seen as the responsibility of only IT teams and BI specialists. However, this is no longer the case as data becomes increasingly essential to modern business decisions.
All members of a business should be educated on why data quality is important. This includes understanding how to maintain data accuracy in the course of their work.
Modern data quality tools can help employees clean and manage data, making it easier to maintain accuracy. It's essential that employees are taught how to use these tools effectively.
Worth a look: How Do You Send an Important Message on Teams
Emerging Challenges
Organizations are facing new data quality issues due to the widespread adoption of cloud computing and big data initiatives. This has led to the inclusion of unstructured and semi-structured data in their systems.
Many organizations now work with both on-premises and cloud systems, making data management more complex. This is due to the need to integrate and manage data from different sources.
The implementation of real-time data streaming platforms is also contributing to data quality challenges. These platforms continuously funnel large volumes of data into corporate systems.
Data scientists are implementing complex data pipelines to support their research and advanced analytics, which can lead to data quality issues if not properly managed.
The growing use of machine learning and AI technologies is also adding to the complexity of managing data quality.
Benefits of Good Data Quality
Having good data quality is crucial for any business. It saves money by reducing the expenses of fixing bad data and prevents costly errors and disruptions. This frees up resources that can be better spent on more productive tasks.
Accurate data also improves the accuracy of analytics, leading to better business decisions that boost sales, streamline operations, and deliver a competitive edge. Reliable data encourages business users to use analytics tools for decision-making instead of relying on gut feelings or makeshift spreadsheets.
Expand your knowledge: Why Is Digital Analytics Important
Some of the benefits of good data quality include:
- Enabling better decision-making
- Improving productivity
- Developing and preserving brand credibility
- Saving time, money, and other assets
- Providing a competitive advantage
- Increasing profitability
These benefits can be seen in various ways, such as reducing the need to spend time and money finding and fixing errors in the data, increasing sales numbers, and decreasing ad waste.
5 Benefits for Business Operations
Having good data quality is essential for business operations. It enables better decision-making by providing accurate and relevant data to base decisions on.
Businesses can be more confident in their decisions with accurate data, which decreases risk and makes it easier to achieve consistent results.
Good data quality also improves productivity by reducing the time employees spend finding and correcting errors. This frees up more time for employees to work on tasks and projects that are a priority.
With accurate data, businesses can target their audience more effectively, reducing ad waste and increasing sales numbers. This is especially true for publishers, who can use data to determine which types of content are most popular on their site.
See what others are reading: What Are Important Components of a Good Backup Plan
Here are some specific benefits of good data quality for business operations:
- Improved customer service by understanding customer perspectives and preferences
- Competitive advantage by spotting opportunities faster than rivals
- Increased ROI by reducing costs associated with poor data quality
- Improved relationships with customers by gathering data about their preferences and needs
- Increased profitability by crafting effective marketing campaigns and reducing ad waste
Validity
Validity is a crucial aspect of data quality, ensuring that data is collected and presented in a way that's consistent and reliable.
Data validity refers to information that fails to follow specific company formats, rules, or processes, as seen in Example 4. This can compromise data quality, making it difficult to organize and analyze.
For instance, if a customer's birthdate is not entered in the correct format, the data becomes invalid. Many organizations design their systems to reject such information, ensuring that only valid data is collected.
Data validity also refers to how the data is collected, not just the data itself. As explained in Example 5, data is valid if it's in the right format, of the correct type, and falls within the right range.
Here are some examples of data validity:
- Using 24-hour time and two digits for minutes and hours, such as 14:34, 17:05, and 08:42, ensures data validity.
- Data that doesn't follow this format, like 2:45, would be invalid.
By ensuring data validity, organizations can avoid the hassle of dealing with invalid data and focus on making informed decisions with reliable information.
Data Quality Management
Data quality management is crucial to ensure the accuracy and reliability of data. Human error is a common cause of inaccuracies in data, and even with spell checking and validation rules, mistakes can still occur.
To mitigate this risk, organizations can implement data quality management tools that automate tasks and procedures using machine learning and other AI technologies. These tools can match records, delete duplicates, and validate new data, making it easier to maintain data integrity.
Data quality managers and data stewards can use collaboration and workflow tools to oversee specific data sets and ensure that data is handled correctly. By establishing employee and interdepartmental buy-in, setting clear metrics, and ensuring high data quality through data governance, organizations can maintain data quality continuity.
Here are some key data quality management best practices to consider:
- Establish employee and interdepartmental buy-in across the enterprise.
- Set clearly defined metrics.
- Ensure high data quality with data governance by establishing guidelines that oversee every aspect of data management.
- Create a process where employees can report any suspected failures regarding data entry or access.
By following these best practices, organizations can ensure that their data quality management system is robust and effective, reducing the risk of human error and maintaining the integrity of their data.
Easier Implementation
Having quality data at your fingertips is a game-changer for any company. Quality data makes it easier to implement insights and make decisions, freeing up time for employees to focus on high-priority tasks.
If your data is incomplete or inconsistent, you'll spend a significant amount of time fixing it, taking away from other activities. This means it takes longer to implement insights and make progress.
Quality data helps keep departments on the same page, allowing them to work together more effectively. This leads to a more efficient and productive business overall.
With fewer inaccuracies in your data, employees will have less time spent finding and correcting errors, giving them more time to focus on what matters. This is a simple yet powerful reason why accurate data makes a big difference.
Broaden your view: Why Is Learning and Development Important for Employees
What Are the Six Elements?
Data quality is a critical aspect of data management, and it's essential to understand the six elements that make up high-quality data. Accuracy is one of these elements, and it refers to the data correctly representing the entities or events it's supposed to represent.
Explore further: When Communicating It's Important to
Data consistency is another important element, which means the data is uniform across systems and data sets, with no conflicts between the same data values in different systems or data sets. This is crucial in ensuring that data is reliable and trustworthy.
Validity is also a key element, as it refers to the data conforming to defined business rules and parameters. Completeness is another essential element, which means the data includes all the values and types of data it's expected to contain, including any metadata that should accompany the data sets.
Data timeliness is also vital, as it refers to the data being current (relative to its specific requirements) and available to use when it's needed. Finally, uniqueness is the last element, which means the data does not contain duplicate records within a single data set, and every record can be uniquely identified.
Here are the six elements of data quality in a concise table:
By understanding and implementing these six elements, organizations can ensure that their data is reliable, trustworthy, and meets its intended purpose.
Timeliness
Timeliness is a crucial aspect of data quality, and it's essential to understand its importance. Data should be recorded as soon as possible after the real-world event it represents occurs.
Data that's outdated can lead to inaccurate results and actions that don't reflect the current reality. For instance, if you have information on customers from 2008, it's an issue with both timeliness and completeness.
Timeliness can have a significant effect on the overall accuracy, viability, and reliability of the data. It's like trying to navigate with a map that's several years old – it's unlikely to get you to your destination accurately.
Data that's current and available when needed is essential for making informed decisions. Timeliness is often overlooked, but it's a critical dimension of data quality.
Here are the six dimensions of data quality, with timeliness being one of them:
- Accuracy: The data correctly represents the entities or events it is supposed to represent.
- Consistency: The data is uniform across systems and data sets.
- Validity: The data conforms to defined business rules and parameters.
- Completeness: The data includes all the values and types of data it is expected to contain.
- Timeliness: The data is current (relative to its specific requirements) and is available to use when it's needed.
- Uniqueness: The data does not contain duplicate records within a single data set.
Data Quality Tools and Practices
Data quality tools and practices can help streamline data management efforts and ensure data integrity. Many organizations use data quality management tools, which can match records, delete duplicates, and validate new data.
These tools often include augmented data quality functions that automate tasks and procedures through machine learning and AI technologies. Centralized consoles or portals allow users to create data handling rules, identify data relationships, and automate data transformations.
Data quality managers and stewards can also use collaboration and workflow tools to oversee data sets and establish shared views of the organization's data repositories. By adopting best practices, such as setting clearly defined metrics and ensuring high data quality, organizations can maintain data quality continuity.
Some key best practices include:
- Establishing employee and interdepartmental buy-in across the enterprise.
- Setting clearly defined metrics.
- Ensuring high data quality with data governance.
- Creating a process for reporting suspected data entry or access failures.
Installing systems to check for common input errors, such as spell checking and validation rules, can also help reduce inaccuracies in data.
Manual Entry
Manual Entry is a common source of inaccuracies in data, and no matter how careful someone is, human error can still occur.
Human error increases with the more data a person has to manage and with the number of people who can access and edit data.
No system is completely immune to human error, so it's essential to test data entry checks regularly to ensure they work properly.
Broaden your view: Why Is Margin of Error Important
Management Tools & Best Practices
To ensure the integrity of your data quality management system, you'll want to consider implementing the right tools and best practices. Organizations often turn to data quality management tools to streamline their efforts, which can match records, delete duplicates, validate new data, and establish remediation policies.
Data quality management tools can also perform data profiling, which examines, analyzes, and summarizes data sets. Some tools even include augmented data quality functions that automate tasks and procedures through machine learning and other AI technologies.
Data quality managers and data stewards might use collaboration and workflow tools that provide shared views of the organization's data repositories and enable them to oversee specific data sets. These tools can also play a role in the organization's master data management (MDM) initiatives.
To maintain data quality continuity, your organization can adopt certain best practices. Here are some key measures to consider:
- Establish employee and interdepartmental buy-in across the enterprise.
- Set clearly defined metrics.
- Ensure high data quality with data governance by establishing guidelines that oversee every aspect of data management.
- Create a process where employees can report any suspected failures regarding data entry or access.
- Establish a step-by-step process for investigating negative reports.
- Launch a data auditing process.
- Establish and invest in a high-quality employee training program.
- Establish, maintain, and consistently update data security standards.
- Assign a data steward at each level throughout your company.
- Leverage potential cloud data automation opportunities.
- Integrate and automate data streams wherever possible.
How Lotame Can Help
Lotame's end-to-end data collaboration platform (DCP) can make onboarding, connecting, enriching, and activating data from various sources a breeze.
This platform helps you organize and integrate data from multiple sources, allowing you to build effective marketing campaigns and other initiatives.
Using Lotame's superior technology to collect data can ensure that your first-party data is high-quality.
Combining this technology with Lotame Precision Audiences can also guarantee that your third-party data is high-quality.
Take a look at this: Why Is First Party Data Important
Data Quality in Specific Contexts
Data quality is crucial in various contexts, including marketing. With high-quality data, marketers can more accurately determine their target audience by collecting data about their current audience and finding potential new customers with similar attributes.
For example, improved audience targeting is a direct result of data quality. Without it, marketers are forced to try to apply to a broad audience, which is not efficient.
Check this out: Why Is Audience Analysis Important
Audience Targeting and Marketing Efforts
Accurate data on your company's customers makes it easier for your marketing team to know exactly what your target audience is.
With high-quality data, you can more accurately determine who your target audience should be by collecting data about your current audience and finding potential new customers with similar attributes.
For more insights, see: Why Is a Target Market Important
Trying to apply to a broad audience without high-quality data is not efficient and can lead to guessing who your target audience should be.
Collecting data about your current audience and using it to find potential new customers with similar attributes can help you more accurately target advertising campaigns.
You can develop products or content that appeal to the right people by using knowledge about your target audience, which is informed by high-quality data.
Accurate data helps your business expand its advertising efforts by appealing to consumers with similar traits to those in your core customer base.
See what others are reading: Why Is Audience Important in Writing
Purchasing
Purchasing data can be a bit tricky, but it's essential to get it right.
First-party data is collected directly from your customers and is generally considered high-quality, as you can be reasonably confident in its accuracy.
You can purchase second-party data from another organization's first-party data, usually via a data collaboration platform. Lotame's second-party data collaboration solutions can help you find reliable data collaboration partners.
A different take: The Most Important Aspect S of a Company's Business Strategy
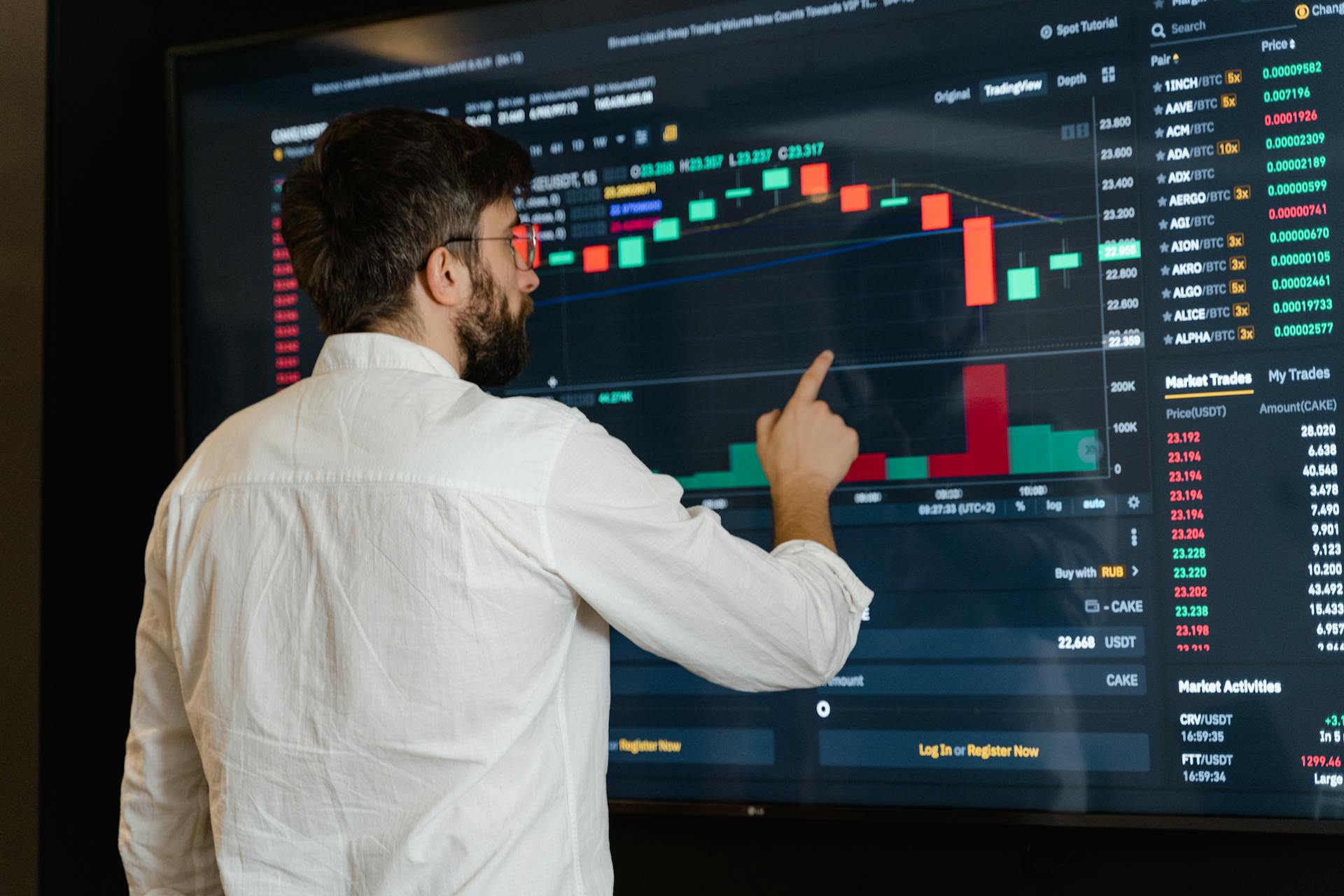
Second-party data is useful for increasing your audience and expanding into new markets. Its quality is usually high, but you need to ensure you're buying it from a reputable company.
Third-party data, on the other hand, is collected from sources that aren't its original collectors and can be purchased from aggregators like the Lotame Data Exchange. It's excellent for expanding your audience due to its volume.
However, third-party data can be harder to verify its accuracy, so it's essential to choose a provider with a good reputation and find out everything you can about the source of the data before purchasing it.
Lotame offers Precision Audiences, which are data segments that exceed industry benchmarks for accuracy and precision. These audiences have been validated using proprietary "continually on" tests, ensuring they are accurate and precise.
You might enjoy: Three Important Things to Know about Your Audience Are
Frequently Asked Questions
What is the importance of quality of information?
Accurate information is crucial for making informed decisions and avoiding misunderstandings. Evaluating the quality of information helps you make reliable choices and build trust in the information you rely on.
What is the impact of data quality?
Maintaining high-quality data helps prevent operational errors, reduces expenses, and boosts revenue. Good data quality also enhances the accuracy of analytics and AI-driven insights
Sources
- https://www.safegraph.com/guides/why-accurate-data-is-important
- https://www.techtarget.com/searchdatamanagement/definition/data-quality
- https://symbio6.nl/en/blog/why-is-data-quality-important
- https://www.alation.com/blog/what-is-data-quality-why-is-it-important/
- https://www.lotame.com/why-is-data-quality-important/
Featured Images: pexels.com