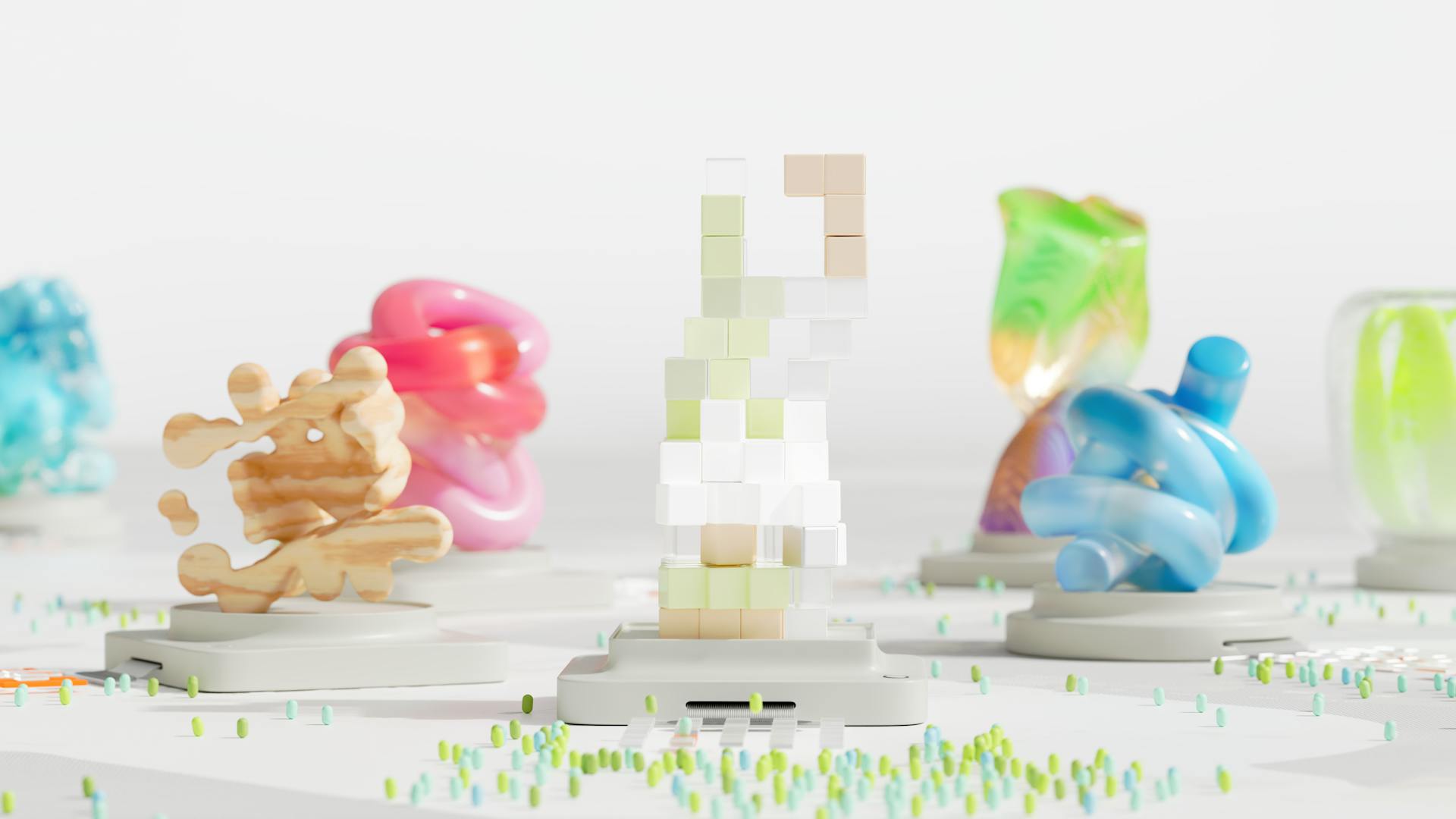
In today's world, responsible AI practices are crucial for an organization's success. This is because AI systems can perpetuate and amplify existing biases, leading to unfair outcomes.
For instance, a study found that AI-powered hiring tools can perpetuate racial and gender biases, resulting in discriminatory hiring practices. This can have severe consequences for both the organization and the individuals affected.
Organizations that prioritize responsible AI practices can avoid these issues and build trust with their customers and stakeholders. By doing so, they can establish a reputation as a responsible and ethical business.
Responsible AI practices also help organizations avoid costly lawsuits and reputational damage.
Check this out: Why Artificial Intelligence Is Important
Fairness and Inclusiveness
Fairness and inclusiveness are crucial aspects of responsible AI practices. AI systems should treat everyone fairly and avoid affecting similarly situated groups of people in different ways.
For example, when AI systems provide guidance on medical treatment, loan applications, or employment, they should make the same recommendations to everyone who has similar symptoms, financial circumstances, or professional qualifications.
Consider reading: Why Is Ai Important to the Future
Inclusion involves incorporating perspectives from a wide range of stakeholders, like experts in ethics and diversity, as well as communities directly and indirectly impacted by the AI system throughout the system's lifecycle.
Google developed an AI system to detect diabetic retinopathy, but it rejected about 20% of all scans when used by practitioners in real-world environments because it was programmed to discard any scans that didn’t meet a specific quality standard.
Involving system users from the start would have identified and resolved these issues much earlier, demonstrating the importance of inclusion in AI development.
Fairness ensures that AI systems' outcomes are equitable and do not discriminate against any groups, particularly those most vulnerable to the harm that can come from AI.
Incorporating fairness into the Responsible AI framework involves a comprehensive approach, including ensuring the Inclusion principle is working well, scrutinizing training data for biases, continually testing for unfair outcomes, and modifying models to address these biases.
The entire goal of fairness is to not harm any vulnerable group, which involves careful design, implementation, and use of AI components to promote equity and reduce bias.
Organisations have a moral obligation to do everything in their power to proactively mitigate the risk of unfair outcomes and the perpetuation of discrimination against already-marginalised groups within society.
Readers also liked: Why Database Management System Is Important
Ensuring Accountability and Trust
Accountability is a crucial aspect of responsible AI practices, and organizations must ensure that those who design and deploy AI systems are held accountable for their actions.
To achieve this, organizations should draw upon industry standards to develop accountability norms, which can ensure that AI systems are not the final authority on any decision affecting people's lives.
Azure Machine Learning provides several MLOps capabilities to increase the efficiency of AI workflows and ensure accountability, including the ability to register, package, and deploy models, capture governance data, and notify and alert on events in the machine learning lifecycle.
The Responsible AI scorecard in Azure Machine Learning also creates accountability by enabling cross-stakeholder communications and empowering developers to configure, download, and share their model health insights with technical and non-technical stakeholders.
To build trust, organizations must demonstrate a commitment to responsible AI practices and provide clear documentation of their AI systems, including data sources, algorithms used, and the decision-making process.
Transparency is a two-sided principle that pairs disclosure elements with explainability, and organizations must carefully consider the amount of disclosure and the type of explanation stakeholder groups should receive.
By prioritizing accountability and transparency, organizations can build trust with stakeholders, including customers, partners, and employees, and create a culture of collaboration and innovation.
Here are some key principles to ensure accountability and trust in AI:
- Develop accountability norms based on industry standards.
- Use MLOps capabilities to increase the efficiency of AI workflows.
- Provide clear documentation of AI systems, including data sources and algorithms used.
- Empower developers to configure, download, and share model health insights.
- Carefully consider the amount of disclosure and the type of explanation stakeholder groups should receive.
Reliability
Reliability is a critical aspect of ensuring accountability and trust in AI systems. It's about building trust by operating reliably, safely, and consistently.
To achieve this, AI systems should be able to operate as designed, respond safely to unexpected conditions, and resist harmful manipulation. This reflects the range of situations and circumstances that developers anticipated during design and testing.
Developers can use the error analysis component of the Responsible AI dashboard to identify cohorts of data with a higher error rate than the overall benchmark. This helps to understand how failure is distributed for a model.
A unique perspective: Why Is Margin of Error Important
Understanding and planning for edge cases is a key area of reliability. Edge cases are situations or conditions that the system or model hasn't been trained for, and can lead to unexpected behavior.
Here are some key areas of reliability in Responsible AI:
- Understanding and planning for edge cases.
- Tracking and adapting to drift in use cases or data.
- Preparing for potential attacks and system obsolescence.
An unreliable AI system can erode trust, hurt users or society at large, and even damage a company's brand or reputation. This is especially true for high-impact use cases like mortgage loan approvals, autonomous vehicles, or medical chatbots.
Building Trust
Building trust is crucial for organizations to harness the full potential of AI. Only one in two people believe that the benefits of AI outweigh the risks, as found in a February 2023 report co-authored by KPMG and the University of Queensland.
Establishing trust with stakeholders is paramount, as it can have far-reaching consequences on a holistic level and individual organizational levels. Organizations can attract customers, investors, and partners who value ethical and socially responsible principles by demonstrating a commitment to responsible AI practices.
Intriguing read: Why Trust Is Important in the Workplace
Building and nurturing trust within the AI ecosystem is a fundamental aspect of responsible AI. By prioritizing trust, organizations can deliver benefits on societal, commercial, and collaborative levels.
Transparency is a key aspect of building trust. Organizations should provide clear documentation of their AI systems, including data sources, algorithms used, and the decision-making process. AI models should be designed to provide human-readable explanations for their decisions.
The Responsible AI dashboard in Azure Machine Learning enables data scientists and developers to generate human-understandable descriptions of the predictions of a model. This includes global explanations, local explanations, and model explanations for a selected cohort of data points.
Here are some key aspects of transparency in AI:
- Global explanations: What features affect the overall behavior of a model?
- Local explanations: Why was a customer's loan application approved or rejected?
- Model explanations: What features affect the overall behavior of a model for low-income applicants?
By providing transparency and explainability, organizations can build trust with their stakeholders and demonstrate a commitment to responsible AI practices.
Meeting Regulatory Requirements
Meeting regulatory requirements is a crucial aspect of responsible AI practices. Organizations must comply with regulations such as the EU AI Act, which can impose significant fines, up to €40 million or 7% of global turnover, if they fail to comply with its provisions.
For more insights, see: Nextjs Ai
To avoid these repercussions, organizations should adopt a framework that incorporates responsible AI principles, which aligns with the EU AI Act's emphasis on transparency and safeguarding individual rights. This approach not only mitigates legal risks but also helps organizations align with societal expectations for ethical and trustworthy AI development and use.
Organizations can position themselves on the right path to meet emerging regulatory standards by embracing responsible AI principles, which can positively impact their reputation and public perception. By doing so, they can earn the trust and confidence of customers, investors, and stakeholders.
Here are some key regulatory requirements to consider:
- Require transparency about data collection, use, and storage.
- Mandate consumer controls to choose how their data is used.
- Implement robust data protection measures, such as anonymization and encryption.
- Obtain explicit user consent for data usage in AI training.
Complying with Regulations
Complying with regulations is a crucial aspect of meeting regulatory requirements. The EU AI Act, currently in the final stages of the legislative process, holds organisations liable for significant fines, reaching up to €40 million or 7% of their global turnover, if they fail to comply with its provisions.
This legislation is just one of many examples, signalling an upcoming deluge of new regulations spanning almost every jurisdiction in the coming years. Organisations must proactively implement responsible AI principles to avoid potential financial and reputational repercussions.
A framework that incorporates responsible AI principles is essential for achieving regulatory compliance. This approach not only mitigates legal risks but also helps organisations align with societal expectations for ethical and trustworthy AI development and use.
By embracing responsible AI, organisations position themselves on the right path to meet emerging regulatory standards. Beyond mere compliance, it demonstrates a commitment to responsible and accountable AI practices, which can positively impact their reputation and public perception.
Organisations that actively prioritise responsible AI stand to gain a competitive edge by earning the trust and confidence of customers, investors, and stakeholders.
Here are some key regulatory measures to consider:
- Transparency and safeguarding individual rights
- Collecting only necessary data
- Ensuring data quality and representativeness
- Maintaining transparency in data collection practices
- Implementing robust security measures to protect data
Sustainability in
Sustainability in AI development is crucial to minimize negative impacts on the environment and those who create these systems. This involves a balance between costs and business value, including environmental impact and human efforts.
High-quality data is essential to reduce the quantity and labor needed for training, making the process more efficient.
Selecting the most efficient models given the requirements is also a key aspect of sustainable AI practices.
Strategically timing the operation of AI systems to align with off-peak and typically cheaper energy periods can also help contain costs.
Human intervention is necessary in various phases of the system's lifecycle, and it's essential to consider when and how it's necessary to balance costs.
Explore further: Important Necessary
Implement with Holistic
Implementing responsible AI practices is crucial for organisations to adopt and scale AI with confidence.
Holistic AI is at the forefront of responsible AI, empowering organisations to adopt and scale AI with confidence through its dedicated platform and solutions.
Our dedicated platform and solutions empower organisations to adopt and scale AI with confidence.
To find out how we can help your organisation take steps towards external assurance, schedule a call with our expert team.
For more insights, see: Why Is Confidence Important in the Workplace
Understanding the Basics
Responsible AI practices are essential for organizations because they ensure that AI systems are transparent, explainable, and fair.
Bias in AI systems can lead to discriminatory outcomes, which can have serious consequences for individuals and organizations.
According to a study, 60% of AI systems tested showed some level of bias.
Organizations must consider the potential risks and consequences of their AI systems, including data breaches and job displacement.
The European Union's General Data Protection Regulation (GDPR) requires organizations to implement robust data protection measures.
By prioritizing responsible AI practices, organizations can build trust with their customers and stakeholders, and maintain a positive reputation.
A survey found that 75% of consumers are more likely to trust a company that uses AI responsibly.
Ultimately, responsible AI practices are crucial for organizations to avoid reputational damage and maintain a competitive edge.
In fact, a study found that companies that prioritize responsible AI practices are 2.5 times more likely to achieve business success.
Discover more: Why Is Zero Trust Important
Sources
- https://learn.microsoft.com/en-us/azure/machine-learning/concept-responsible-ai
- https://www.microsoft.com/cms/api/am/binary/RE4pKH5
- https://ai.google/responsibility/responsible-ai-practices/
- https://www.holisticai.com/blog/3-reasons-organisation-needs-responsible-ai
- https://www.neudesic.com/blog/understand-responsible-ai/
Featured Images: pexels.com