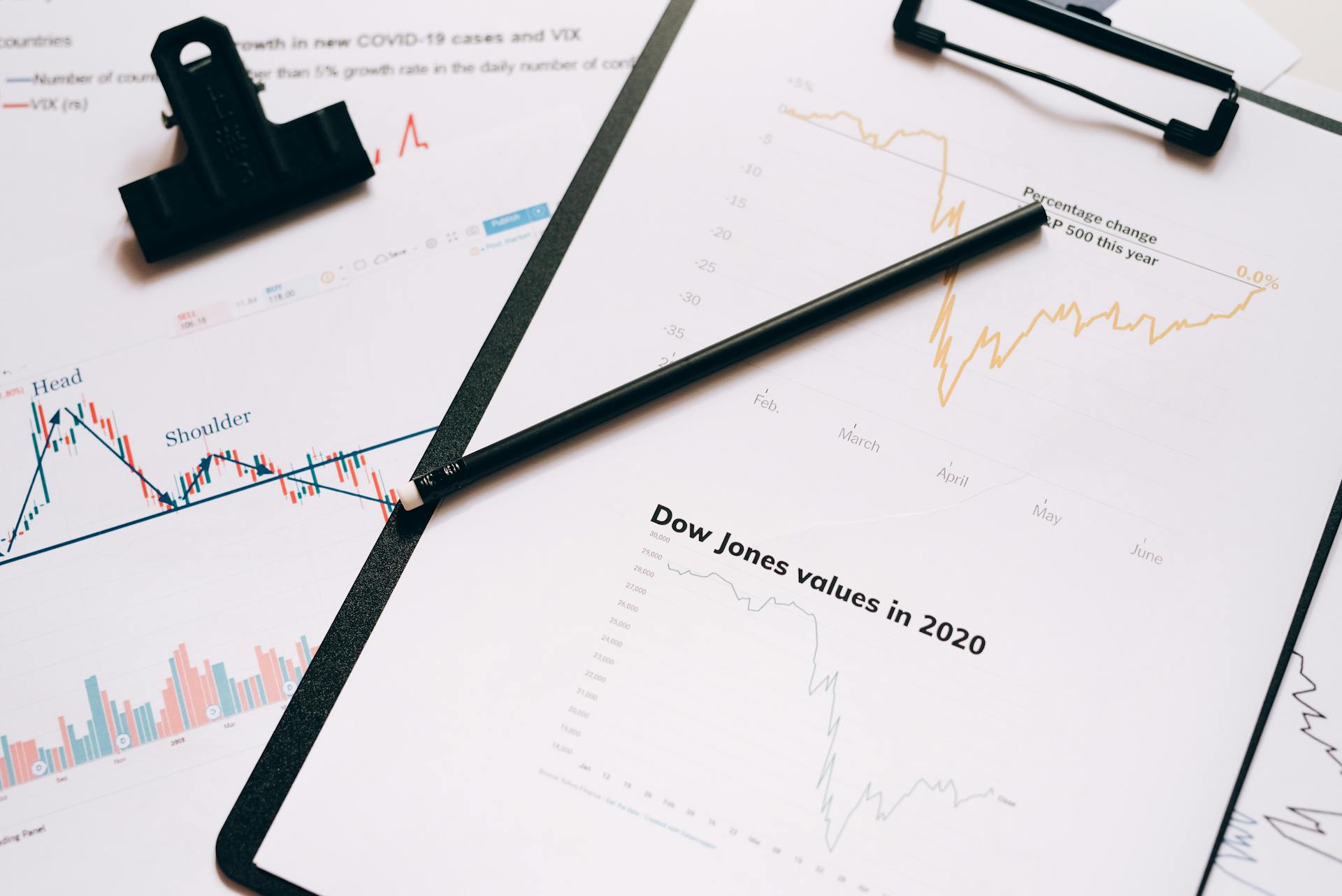
Using statistical analysis can help businesses make informed decisions, as seen in the example of a company that increased sales by 25% after analyzing customer purchasing patterns.
By identifying statistically significant trends, companies can adjust their strategies to capitalize on opportunities and mitigate risks.
A well-structured statistical model can reveal valuable insights, such as the correlation between product features and customer satisfaction.
This can lead to improved product development and customer experience, ultimately driving business growth.
On a similar theme: Why Is Product Planning Important
What Is Statistically Important?
Statistical significance is not the same as clinical significance. Statistical significance is determined by mathematical formulas, like a p value, but it doesn't necessarily mean the results are important to patients or clinicians.
To determine clinical significance, healthcare providers need to use their knowledge and experience to evaluate the study's design, sample size, power, and data analysis. This is because a study with statistically significant results and a large sample size may not be clinically significant, while a study with smaller sample size and non-significant results could still be important in practice.
In fact, some experts argue that the terms "statistically significant" or "not significant" should be excluded from research reports because they don't accurately convey the practical importance of the findings.
For more insights, see: Significant Important
Definition/Introduction
Statistical significance is a crucial concept in various fields, including medicine and experimentation. It's often misunderstood, but understanding it can help you make informed decisions.
To determine statistical significance, you need to consider the sample size and the number of variations in an experiment. With a large sample size and fewer variations, you're more likely to get accurate results.
Stats Engine, a statistical model built-in to Optimizely, helps you determine the practical significance of your experiments. It combines sequential testing and false discovery rate control to give you trustworthy results faster.
Real-time monitoring of results is possible with Stats Engine, allowing you to adjust your testing and make decisions without sacrificing data integrity. This is especially useful in medical research, where evidence-based medicine guides decision-making.
Statistical significance can be increased over time as more evidence is collected. This evidence comes in two forms: larger conversion rate differences and conversion rate differences that persist over more visitors.
Clinical
Clinical significance is a crucial concept in evidence-based medicine that goes beyond statistical significance. Healthcare providers need to consider the practical importance of study results through careful evaluation of the design, sample size, power, likelihood of type I and type II errors, data analysis, and reporting of statistical findings.
A study with statistically significant differences and a large sample size may be of no interest to clinicians, whereas a study with a smaller sample size and statistically non-significant results could still impact clinical practice. This highlights the importance of using clinical judgment to determine the meaningfulness of study results.
The decision on what is clinically significant can be challenging, depending on the providers' experience and especially the severity of the disease. Providers should use their knowledge and experiences to determine the meaningfulness of study results and make inferences based not only on significant or insignificant results by researchers but through their understanding of study limitations and practical implications.
A fresh viewpoint: Why Is It Important to Study Communication
Statistical significance determines if there is mathematical significance to the analysis of the results, but clinical significance means the difference is vital to the patient and the clinician. For example, a 10 mmHg drop in systolic blood pressure may be clinically significant, but a 10-minute increase in life expectancy may not be.
Two studies can have a similar statistical significance but vastly differ in clinical significance, as seen in the example of two new chemotherapy agents for treating cancer. Drug A increased survival by at least 10 years with a P value of 0.01 and an alpha for the study of 0.05, making it both statistically and clinically significant. In contrast, Drug B increased survival by at least 10 minutes with a P value of 0.01 and alpha for the study of 0.05, making it statistically significant but not clinically significant.
See what others are reading: 10 Most Important Metrics for Web App Monitoring
Why Is Statistical Importance Important to Businesses?
Statistical significance is crucial for businesses to make informed decisions based on data. It helps you understand how strongly the results of your surveys, experiments, polls, or user data should influence your decisions.
A larger sample size generally provides more reliable results. For website tests, more traffic means quicker, more accurate results. This is why having a bigger audience for your tests is beneficial.
Random sampling is essential for bridging the statistically significant difference and getting accurate results. If you don't distribute your test variations randomly among your audience, you might introduce bias.
Statistical significance helps you distinguish between real improvements and random chance. This is especially important in industries like pharmaceuticals, where it can determine a drug's effectiveness and influence investor funding.
Here are the two key factors that determine statistical significance:
- Sample size: The number of participants in your experiment.
- Effect size: The magnitude of difference between your test variations.
These factors work together to ensure that your results are reliable and accurate, giving you confidence in your analysis and its resulting insights.
Calculating Statistical Importance
Calculating statistical importance is crucial for making informed decisions based on data. It helps businesses distinguish between real improvements and random chance.
Statistical significance is often calculated with statistical hypothesis testing, which tests the validity of a hypothesis by figuring out the probability that your results have happened by chance. A standard hypothesis test relies on two hypotheses: the null hypothesis and the alternative hypothesis.
For more insights, see: Why Is Statistical Significance Important
The null hypothesis is the default assumption of a statistical test that you're attempting to disprove, while the alternative hypothesis is an alternate theory that contradicts your null hypothesis. For example, if you're testing whether an increase in cost will affect the number of purchases, the null hypothesis would be that an increase in cost won't affect the number of purchases.
Two key factors influence statistical significance: sample size and effect size. A larger sample size generally provides more reliable results, while a larger effect size shows how much impact your changes have made. Random sampling is crucial for bridging the statistically significant difference and getting accurate results.
Here's a quick rundown of the key factors to consider:
To get valid results from experiments, you need to set a minimum detectable effect and sample size in advance, avoid peeking at results, and not test too many goals or variations at the same time. With Stats Engine, you can easily determine the practical significance of your experiments and get trustworthy results faster.
Understanding Statistical Significance
Statistical significance is a crucial concept in research, but it can be tricky to understand. A P value less than a predetermined alpha is considered a statistically significant result.
The alpha value, denoted by the Greek letter alpha (α), is the maximum P-value we can accept to consider a study statistically significant. In most sciences, results yielding a P-value of 0.05 are considered on the borderline of statistical significance.
To determine if a result is statistically significant, we need to consider the P-value and the significance level. A P-value of 0.05 means there's a 5% chance of the results occurring randomly, while a P-value of 0.01 means there's a 1% chance.
Here's a quick reference guide to P-values and their corresponding probabilities:
- P-value of 0.05: 5% chance of results occurring randomly
- P-value of 0.01: 1% chance of results occurring randomly
- P-value of 0.005: 0.5% chance of results occurring randomly
By understanding P-values and significance levels, we can better evaluate the results of a study and determine if they're statistically significant.
Improving Healthcare Outcomes
Having a cohesive understanding of statistical significance is crucial for healthcare teams to make informed decisions. If team members don't grasp this concept, they may draw opposing conclusions from the same research.
Healthcare team members, including nurses, physicians, and pharmacists, rely heavily on literature reviews to inform their practice. Without a shared understanding of statistical significance, team members may misinterpret research findings.
A harmonious understanding of statistical significance is essential for healthcare teams to provide high-quality care. Without it, team members may make decisions that put patients at risk.
In healthcare, statistical significance is not just a theoretical concept, it has real-world implications. Healthcare teams need to understand its importance to make informed decisions that improve patient outcomes.
Consider reading: Why Is Team Development Important
Z-Score
A Z-score is the number of standard deviations a data point is distanced from the mean.
It's calculated by subtracting the mean from the value of the data point and dividing the result by the standard deviation. The formula is Z = (x – μ)/(σ).
MixTunes would have a Z-score of 2 since the mean is 1000 downloads and the standard deviation is 100 downloads.
For another approach, see: Why Standard Is Important
The magnitude of the Z-score determines how meaningful the result is. The higher the magnitude, the more unlikely the result is to happen by chance.
A higher Z-score indicates a more meaningful result, but it's not the only factor to consider. The Z-score formula is slightly changed for studies with a sample of the overall population.
Expand your knowledge: What Is Meaningful Use and Why Is It Important
Significance
Statistical significance is a crucial concept in research, and it's essential to understand how it works. A P-value less than a predetermined alpha is considered a statistically significant result.
The alpha level, denoted by the Greek letter alpha (α), is the maximum P-value we can accept to consider the study statistically significant. In most sciences, results yielding a P-value of .05 are considered on the borderline of statistical significance.
A low P-value means that the observed result is unlikely to occur by chance alone, making it a statistically significant finding. For example, if the P-value is under .01, results are considered statistically significant, and if it's below .005, they are considered highly statistically significant.
Explore further: When Communicating It's Important to
To determine statistical significance, we need to consider the sample size and the effect size. A larger sample size and a larger effect size increase the chances of a statistically significant finding.
Here's a rough guide to understanding P-values:
Keep in mind that statistical significance does not necessarily mean practical significance. A study can be statistically significant but have no practical implications.
Best Practices for Statistical Analysis
To get always valid results from experiments, you need to follow some strict guidelines. These guidelines include setting a minimum detectable effect and sample size in advance, not peeking at results, and not testing too many goals or variations at the same time.
To simplify this process, you can use Stats Engine, which calculates the estimated duration of your experiment with just two formulas: Total visitors needed = Sample size × Number of variations, and Estimated days to run = Total visitors needed ÷ Average daily visitors.
Stats Engine operates by combining sequential testing and false discovery rate control, allowing for real-time monitoring of results, adaptive testing that adjusts to true effect size, and faster decision-making without sacrificing data integrity.
Best Practices for Reaching
To reach statistical significance, it's essential to design experiments with significant potential impact on user behavior. This will give you a better chance of detecting meaningful differences.
Running tests for at least one business cycle (7 days) is crucial. This allows you to collect enough data to make informed decisions.
Choosing primary and secondary metrics carefully is vital. Select metrics that are relevant to your experiment and will provide valuable insights.
Here are some key considerations to keep in mind:
- Run tests for at least 7 days
- Choose primary and secondary metrics carefully
- Design experiments with significant potential impact on user behavior
Get Valid Results with Stats Engine
Stats Engine is a game-changer for anyone working with statistical analysis. It's an advanced statistical model built-in to Optimizely that helps you get always valid results from your experiments.
To get valid results, you need to follow a strict set of guidelines, but Stats Engine makes it easy. You can calculate the estimated duration of your experiment using just two simple formulas: Total visitors needed = Sample size × Number of variations, and Estimated days to run = Total visitors needed ÷ Average daily visitors.
Stats Engine operates by combining sequential testing and false discovery rate control to give you trustworthy results faster, regardless of sample size and type of data. This means you can have real-time monitoring of results, adaptive testing that adjusts to true effect size, and faster decision-making without sacrificing data integrity.
As you collect more evidence, statistical significance should generally increase over time. This means you'll see larger conversion rate differences and conversion rate differences that persist over more visitors.
Here's a summary of the benefits of using Stats Engine:
- Real-time monitoring of results
- Adaptive testing that adjusts to true effect size
- Faster decision-making without sacrificing data integrity
Statistical Inference
Statistical inference is a crucial aspect of determining statistical significance. It's used to make conclusions about a population based on a sample of data. In hypothesis testing, statistical inference helps determine whether the observed difference between a variation and a baseline is due to chance or a real effect.
A p-value is a key output in statistical inference, indicating the likelihood of seeing evidence as strong or stronger in favor of a difference in performance between a variation and a baseline, assuming there's no real difference.
Intriguing read: Why Is Variation Important
The confidence interval is another important output, providing an estimated range of values that are likely to include the unknown but exact value summarizing the target population if an experiment was replicated numerous times.
To determine statistical significance, you'll want to pay attention to both the p-value and the confidence interval. A low p-value and a narrow confidence interval can indicate that the observed difference is statistically significant.
Here's a simple way to think about it: if your p-value is low, it means that the observed difference is unlikely to be due to chance. But if your confidence interval is wide, it may indicate that the observed difference is not reliable.
In practice, it's essential to follow strict guidelines to get valid results from experiments, such as setting a minimum detectable effect and sample size in advance, not peeking at results, and not testing too many goals or variations at the same time.
Stats Engine is a powerful tool that can help you determine the practical significance of your experiments. It operates by combining sequential testing and false discovery rate control to give you trustworthy results faster, regardless of sample size and type of data.
With Stats Engine, you can calculate the estimated duration of your experiment using the following formula:
Total visitors needed = Sample size × Number of variations
Estimated days to run = Total visitors needed ÷ Average daily visitors
A different take: What Is an Important Difference between Statistics and Parameters
Common Issues and Concerns
Choosing the right alpha level is crucial, as there's nothing special about picking an alpha of 0.05. Historically, researchers have concluded that an alpha of 0.05, or a one in 20 chance of being incorrect, is good enough, but the researcher must consider what the confidence level should genuinely be for the research question being asked.
A smaller alpha, such as 0.01, may be more appropriate in certain situations. It's essential to specify the alpha level before any intervention or collection of data, rather than "seeing what the data shows" and then picking an alpha to give a statistically significant result.
Selecting the correct statistical analysis method is equally important, as there are numerous methods for obtaining a P value. The method chosen depends on the type of data, the number of data points, and the question being asked.
Using the wrong statistical method can lead to meaningless results, as an incorrect P value would be calculated. It's crucial to consider these questions during the study design so the statistical analysis can be correctly identified before the research.
Explore further: Why Is Customer Lifetime Value Important
Featured Images: pexels.com