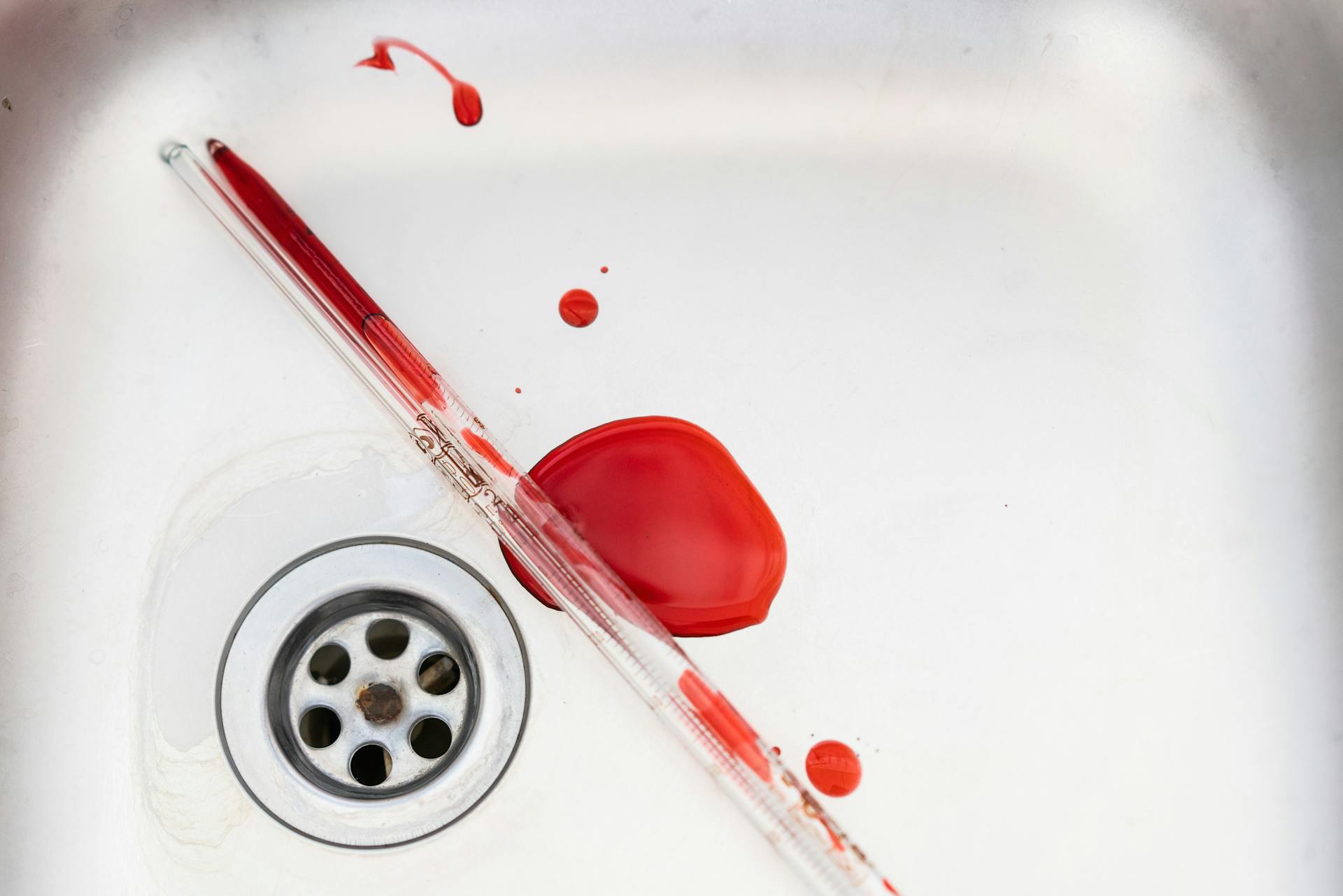
A/B testing is a powerful tool that can make or break a business. By testing different versions of a website, email, or ad, you can identify what works and what doesn't, and make data-driven decisions to improve your bottom line.
According to a study, companies that use A/B testing see an average increase in conversion rates of 18%. This is a significant boost that can make a huge difference in revenue.
A/B testing is not just about trying out new ideas; it's also about refining existing processes. By testing small changes, you can identify areas for improvement and make incremental gains over time.
The key to successful A/B testing is to keep it simple and focused. Don't try to test too many variables at once, as this can lead to confusing results.
For another approach, see: Why Is It Important to Identify Your Prime Time
What Is A/B Testing
A/B testing is a method used to compare two versions of a product, page, or feature to determine which one performs better. This can be done to test anything from website layouts to marketing campaigns.
A/B testing is not just limited to digital products, it can also be used to test physical products. For example, a company might test two different packaging designs to see which one is more appealing to customers.
The goal of A/B testing is to identify which version performs better, whether it's through increased sales, engagement, or other metrics. By doing so, businesses can make data-driven decisions to improve their products and services.
A/B testing can be done with a small sample size, making it a cost-effective way to test and refine ideas. In fact, a study found that even with a small sample size of just 100 users, A/B testing can still provide statistically significant results.
By regularly conducting A/B tests, businesses can avoid making costly mistakes and instead make informed decisions that drive growth and improvement.
For more insights, see: Why Is the Bechdel Test Important
Benefits of A/B Testing
A/B testing is a powerful tool that can bring significant benefits to your website or mobile app.
Running an A/B test can have a significant positive effect on your site or mobile app, making it easy to implement and providing massive returns and valuable learning for your team.
A/B testing helps increase user engagement and conversion rates, while reducing bounce rates and minimizing risk.
The cost of acquiring quality traffic is huge, but A/B testing lets you make the most out of your existing traffic, increasing conversions without spending additional dollars on acquiring new traffic.
A/B testing can give you high ROI, as even the smallest changes on your website can result in a significant increase in overall business conversions.
By improving conversion funnels, data from A/B testing can help businesses maximize existing traffic ROI.
A/B testing helps to identify which changes have a positive impact on UX and improve conversions, making it a cost-effective approach compared to investing in earning new traffic.
Reducing bounce rates is crucial, and A/B testing can help you find friction and visitor pain points, improving your website visitors' overall experience and making them spend more time on your site.
A/B testing is the simplest and most effective means to determine the best content to convert visits into sign-ups and purchases.
A unique perspective: Why Is Site Speed Important
Major decisions can be well-informed, avoiding costly and time-intensive changes that are proven ineffective, and reducing risks by avoiding mistakes that could tie up resources for minimum or negative gain.
Analytics can tell you what to optimize, helping you identify high traffic areas of your site or app and low-converting or high-drop off pages that can be improved.
Testing out different user experience elements through A/B testing is a powerful way for companies to make changes that lead to positive results and learn what's going to be the most effective.
Since A/B testing is entirely data-driven, you can quickly determine a "winner" and a "loser" based on statistically significant improvements in metrics like time spent on the page, number of demo requests, cart abandonment rate, click-through rate, and so on.
Performing a series of A/B tests minimizes the risk of making sweeping changes by first testing a smaller segment of your user base, allowing you to compare the behaviors of the experimental group with the behaviors of your control group to see which version of your product produces the best results.
You might enjoy: Why Is Content Moderation Important for User Generated Campaigns
Planning and Preparation
To plan and prepare for an A/B test, you need to follow a series of steps. These include choosing the element to test, creating a hypothesis, determining the conversion goal, and identifying the control and implementing the change to test.
Before running an A/B test, it's essential to define your business goals and key performance indicators (KPIs). For example, an online mobile phone cover store might aim to increase revenue by increasing online orders and sales, with the KPI being the number of phone covers sold. This helps you understand what you're trying to achieve and measure the success of your test.
To prepare for an A/B test, create a backlog of elements to test based on data analysis. This might include items like adding multiple payment options to reduce drop-off on the checkout page. With a data-backed backlog, you can formulate a hypothesis for each item, such as "adding multiple payment options will help reduce drop-off on the checkout page."
Broaden your view: What Content Is Important to Include in Your Webpage
Make Low-Risk Modifications
Making low-risk modifications is a crucial part of planning and preparation for A/B testing. By making minor, incremental changes to your web page, you can reduce the risk of jeopardizing your current conversion rate.
A/B testing lets you target your resources for maximum output with minimal modifications, resulting in an increased ROI. This approach is especially useful when you plan to remove or update product descriptions, as you can analyze how visitors react to the change.
You can also use A/B testing to introduce new features, launching them as a test to understand whether or not the new change will please your website audience. This approach helps you make data-based conclusions and determine which variation to implement or identify the need for further testing.
Major changes to your website can be risky, as they may not pay off in the short or long run. Instead, A/B testing can help you make small changes rather than implementing a total redesign.
A fresh viewpoint: Making Folders and Filing Important Emails
Here are some benefits of making low-risk modifications:
- Reduced risk of jeopardizing current conversion rate
- Increased ROI through targeted resource allocation
- Data-based conclusions for determining which variation to implement
- Opportunity to test new features and understand audience reaction
By making low-risk modifications, you can systematically work through each part of your website to improve conversions and achieve your business goals.
How to Plan a Calendar
Planning a calendar for A/B testing is crucial to ensure a structured approach. A good amount of brainstorming, along with real-time visitor data, is the way to go about it.
You begin by analyzing existing website data and gathering visitor behavior data. This helps you prepare a backlog of action items based on them.
A good testing calendar or a CRO program will take you through 4 stages.
You might enjoy: What Are Important Components of a Good Backup Plan
Prioritize
Prioritizing your website changes is crucial to maximize your ROI. Limit yourself to one variation per A/B test, as changing multiple variables can lead to unclear results.
To set priorities, analyze your website data and visitor behavior metrics in the planning stage. This will help you identify areas for improvement and create a list of website pages or elements to be changed.
Use frameworks like ICE, PIE, or LIFT to help with the prioritization process. These frameworks consider factors such as competence, importance, and ease of implementation.
By prioritizing your website changes, you can ensure that you're targeting the most impactful areas first. This will help you make the most of your resources and minimize the risk of jeopardizing your current conversion rate.
Recommended read: What Is an Important Factor That Help Determines Cost
Common Mistakes to Avoid
A/B testing demands planning, patience, and precision to avoid costly mistakes.
Making silly mistakes can cost your business time and money, which you can’t afford. A/B tests are designed to test one variable at a time, limiting the A and B choices to a single change to know with certainty that this specific alteration was responsible for the final outcome.
Failing to follow an iterative process is a common mistake, where businesses give up on A/B testing after their first test fails, missing out on the opportunity to draw insights from previous tests and plan for a successful next test.
A different take: Testing-library/jest-dom How to Test with Next Js
What to Avoid
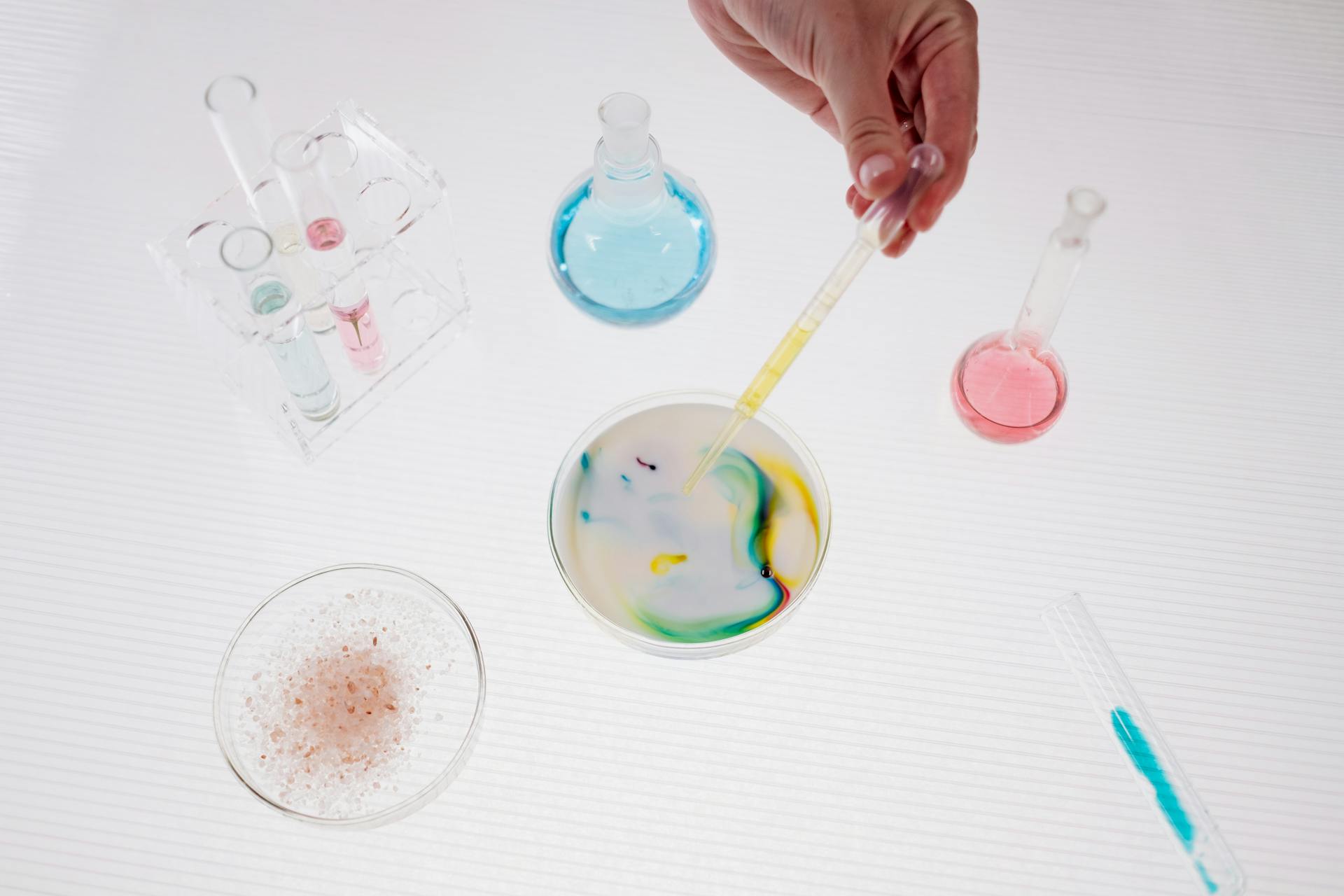
A/B testing is an effective way to move business metrics in a positive direction, but it demands planning, patience, and precision. Making silly mistakes can cost your business time and money.
To avoid making blunders, remember that A/B testing is designed to test one variable at a time. Limiting the A and B choices to a single change allows you to know with certainty that this specific alteration was responsible for the final outcome.
Failing to follow an iterative process is a common mistake. Businesses often give up on A/B testing after their first test fails, but to improve the chances of your next test succeeding, you should draw insights from your last tests while planning and deploying your next test.
Don't stop testing after a successful one. Test each element repetitively to produce the most optimized version of it even if they are a product of a successful campaign.
If this caught your attention, see: Are One Piece Filler Episodes Important
Too Many Elements
Testing too many elements together can be a recipe for disaster. Industry experts warn that running multiple tests at once makes it difficult to pinpoint which element influenced the test's success or failure.
The more elements you test, the more traffic your page needs to justify statistically significant testing. Prioritization of tests is indispensable for successful A/B testing, as noted by industry experts.
Testing too many elements together can dilute the effectiveness of your tests and make it harder to identify what's working and what's not. This can lead to wasted resources and missed opportunities.
Industry experts caution against running too many tests at the same time, as it can make it challenging to pinpoint which element influenced the test's success or failure the most.
A different take: The Most Important Aspect S of a Company's Business Strategy
Incorrect Duration
Running an A/B test for too short or too long a period can be detrimental to its success.
The duration of a test depends on factors like existing traffic, conversion rates, and expected improvement.
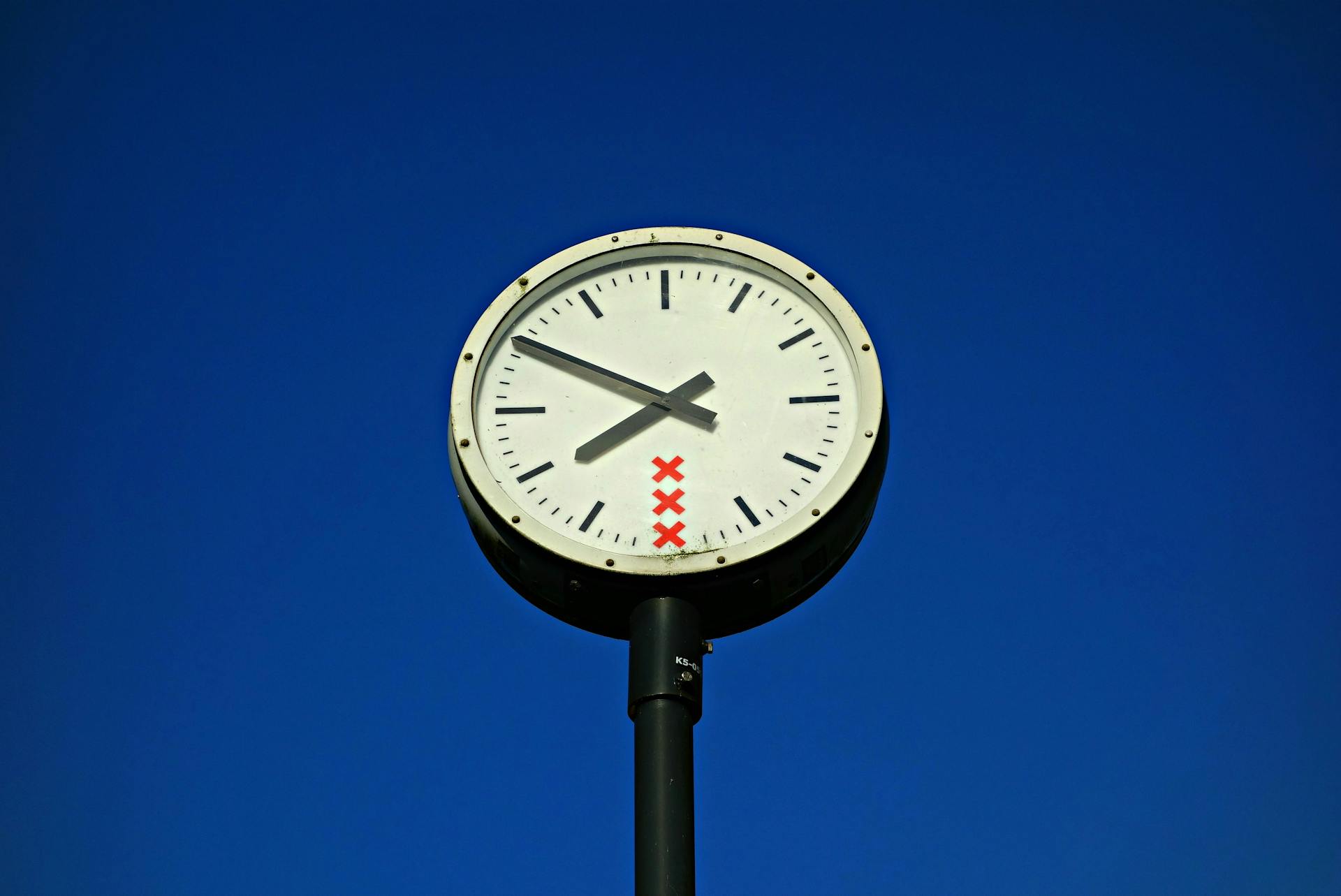
You shouldn't call off a test just because one version appears to be winning early on.
Letting a campaign run for too long is also a mistake businesses often make.
The amount of time required for a reliable test will vary depending on your website's traffic and conversion rates.
A good testing tool should tell you when you've gathered enough data to draw reliable conclusions.
Update your site with the desired variation(s) and remove all test elements as soon as possible.
Choosing the Right Tools and Approach
There are two types of statistical approaches used in A/B testing: Frequentist and Bayesian.
The Bayesian approach is preferred by VWO, and it's used to successfully run an A/B test and draw the right business conclusion.
The choice between these two approaches depends on the specific needs of your experiment, but understanding the pros and cons of each can help you make an informed decision.
Should You Consider
If you're struggling with a high cart abandonment rate, low viewer engagement, or unqualified leads, you should consider A/B testing. A/B testing can help pinpoint the cause of these issues and identify areas for improvement.
Common conversion funnel leaks include confusing calls-to-action buttons, poorly qualified leads, complicated page layouts, and too much friction leading to form abandonment on high-value pages. A/B testing can be used to test various landing pages and other elements to determine where issues are being encountered.
You can use A/B testing to boost the performance of your marketing efforts by identifying the most effective messaging, offers, and design elements. For example, you can test different CTA button colors to see which one produces more clicks.
A/B testing can be used in conjunction with a full website redesign to refine elements within your site and test those. This can help you make data-backed decisions and avoid wasting spend on lesser-performing ads.
Some common elements that can be A/B tested include:
- Headline or subject line
- Imagery
- Call-to-action (CTA) forms and language
- Layout
- Fonts
- Colors
By testing one change at a time, you can see which elements affect users' behavior and which do not. This can help you optimize the user experience and improve engagement.
Choosing a Statistical Approach
Choosing a statistical approach is a crucial step in A/B testing, and it's essential to understand the two main approaches: Frequentist and Bayesian. The Bayesian approach is a theory-based approach that deals with the Bayesian interpretation of probability, where probability is expressed as a degree of belief in an event.
The Frequentist approach, on the other hand, is based on the assumption that there are no differences between A and B, and it only uses data from the current experiment. In contrast, the Bayesian approach uses prior knowledge from previous experiments and incorporates that information into the current data.
The Bayesian approach provides actionable results almost 50% faster while focusing on statistical significance. It also gives you more control over testing, allowing you to plan better, have a more accurate reason to end tests, and get into the nitty-gritty of how close or far apart A and B are.
Here's a comparison table to help you understand the two approaches:
Ultimately, the choice between the Frequentist and Bayesian approaches depends on your specific needs and goals. If you want to achieve statistically significant results faster, the Bayesian approach might be the better choice.
For your interest: Why Is Word Choice Important in Writing
Frequentist Approach
The frequentist approach is a popular method for interpreting A/B testing results. It's based on the assumption that there are no differences between A and B.
A low p-value means there's a high likelihood of differences between the two versions. This approach is fast and has many resources available.
However, it's impossible to get meaningful results until the tests are fully completed. You won't know how much a variation won by, just that it did.
This approach is suitable for businesses that need quick results, but it's essential to keep in mind its limitations.
Readers also liked: What Are Important Differences between Telehealth and in Person Care
Choosing a Run Type
Consider the number of changes you'll be making, the number of pages involved, and the amount of traffic required to get a statistically significant result.
The extent of the problem you're trying to solve is also crucial. For example, a conversion on a landing page that can benefit from changing a button color is a perfect use case for A/B testing.
If this caught your attention, see: What Is an Important Number in Computers
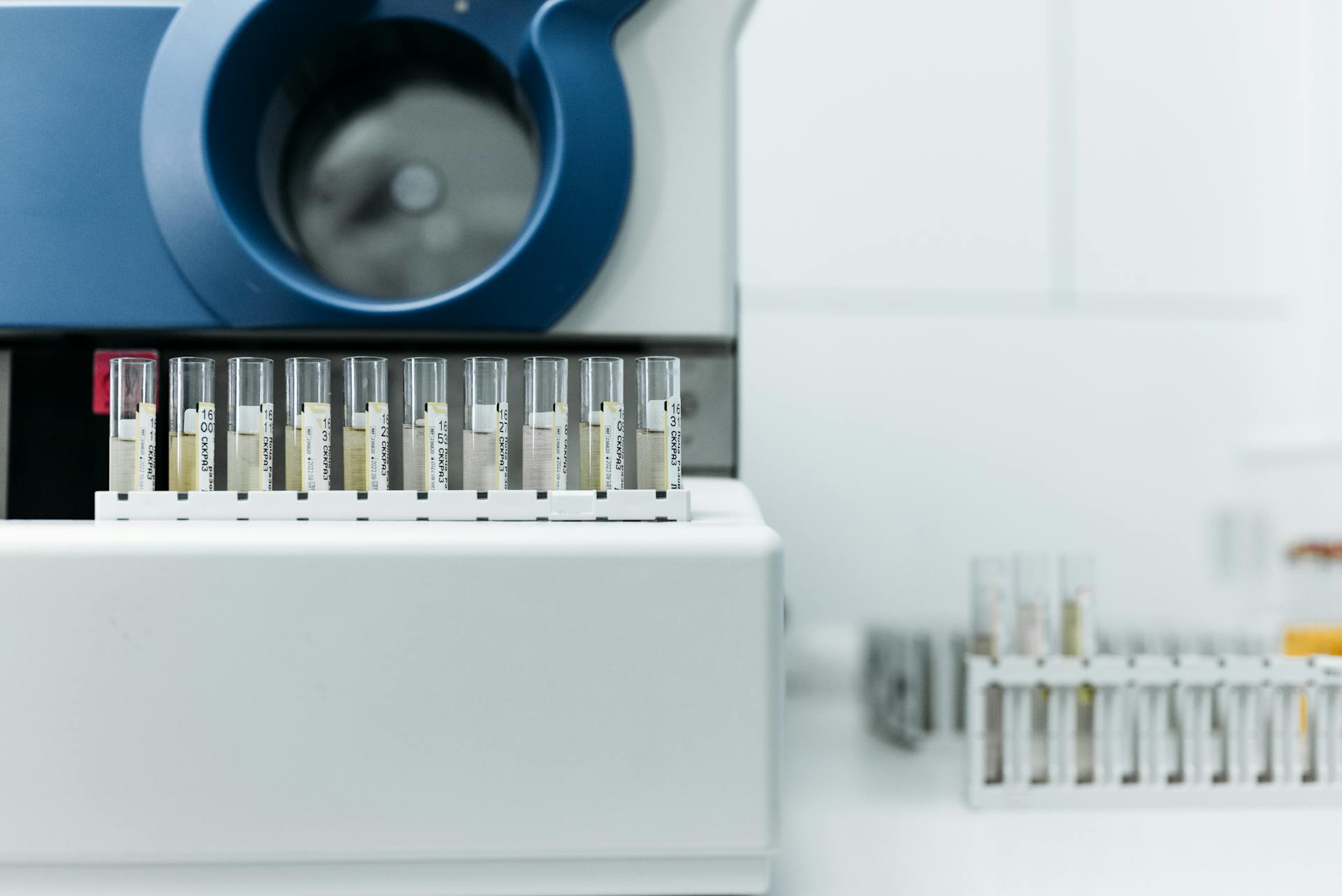
You'll need to think about the complexity of the test. Changing multiple pages that a user encounters across their customer journey is better suited for multipage testing.
Here are the key factors to consider when choosing a run type:
- Number of changes you'll be making
- Number of pages involved
- Amount of traffic required for a statistically significant result
- Extent of the problem you're trying to solve
These factors will help you determine whether to use A/B testing, multipage testing, or another approach.
Server-Side vs. Client-Side
Server-side testing is a more robust approach that allows for the testing of additional elements. For instance, it's ideal for determining whether speeding up page load time increases engagement.
Server-side testing is particularly useful for measuring the response to workflow changes. This type of testing can help you identify areas for improvement in your website's functionality.
Server-side testing gives you more flexibility in terms of what you can test. It's a bit more complex, but it provides more in-depth insights into your website's performance.
Server-side testing can be used to test the impact of various factors on user behavior, such as the effectiveness of different workflows. This can help you make data-driven decisions about your website's design and functionality.
Additional reading: When Communicating It's Important to
Server-side testing can also be used to test the impact of technical changes, such as changes to page load time, on user engagement. This can help you identify areas where you can improve the user experience.
Server-side testing is a powerful tool that can help you gain a deeper understanding of your website's performance and user behavior.
User Segmentation
User segmentation is a crucial step in A/B testing, allowing you to create a subset of specific users based on similar behavioral or demographic traits.
Creating a segment of users who have used your product for fewer than three months can help you understand how design changes affect those who will be using it. This is because customers who have used your product for more than three months are unlikely to use certain features.
Amplitude can build a cohort of customers most likely to perform a certain action in the future, such as using a chatbot. This predictive cohort can help you target users who are actually interested in using a chatbot.
Testing a broad range of customers may dilute the result from the segment you wish to target, making user segmentation essential for accurate A/B test results.
Intriguing read: Why Is Bandwidth Important to Internet Users
Types of A/B Testing
There are four basic testing methods besides conventional A/B testing: A/B testing, Split URL testing, Multivariate testing, and Multipage testing.
A/B testing is a straightforward method that involves testing two versions of a web page element to see which one performs better. For example, you might test two versions of a hero image, call-to-action button color, and headlines on a landing page.
Split URL testing is not explicitly mentioned in the provided article sections, but it's implied to be one of the four basic testing methods. Multivariate testing, on the other hand, involves testing variations of multiple-page variables simultaneously to find the best combination.
Multivariate testing can help eliminate the need to run multiple sequential A/B tests on a web page with similar goals, saving time, money, and effort. It can also help analyze the contribution of each page element to the measured gains and map all the interactions between independent element variations.
For your interest: One Important Purpose of a Brand Is to
Here are the four basic testing methods in a concise table:
Multipage testing, in particular, allows you to create consistent experiences for your target audience by implementing the same change on several pages. This can help ensure that your website visitors don't get distracted and bounce off between different variations and designs.
Address Visitor Pain Points
Addressing visitor pain points is crucial to creating a positive user experience. Visitors come to your website with a specific goal in mind, and if they encounter pain points, it can lead to frustration and a bad user experience.
Common pain points include confusing copy, hard-to-find CTA buttons, and roadblocks that make it difficult to complete their goals. These issues can be solved by using data gathered through visitor behavior analysis tools like heatmaps, Google Analytics, and website surveys.
Heatmaps, for example, can help identify where users are clicking and what they're struggling with. Fullstory is another tool that uses heatmaps, funnel analysis, session replay, and other tools to help teams perfect their digital experiences.
Curious to learn more? Check out: Important Points
By analyzing both quantitative and qualitative data, you can identify the source of user pain points and start fixing them. This approach can help improve conversion rates and create a better user experience.
Here are some common visitor goals and potential pain points:
- Learning more about a deal or special offer: Confusing copy or hard-to-find CTA buttons
- Exploring products or services: Difficulty finding relevant information or navigating the website
- Making a purchase: CTA buttons that don't work or a checkout process that's too complicated
- Reading or watching content about a particular subject: Poorly organized content or difficulty finding relevant articles
By understanding these pain points and addressing them, you can create a more user-friendly experience and improve conversion rates.
Statistical Significance
Statistical Significance is a crucial aspect of A/B testing. It's the claim that a set of observed data are not the result of chance but can instead be attributed to a specific cause.
To determine statistical significance, you need to let your A/B test run through its entire course, so it reaches its statistical significance. This ensures that your results are reliable and meaningful.
A statistically significant result is one that is 95% or higher. However, testing managers might use 90% to achieve results faster, which requires a smaller sample size.
Check this out: Why Is Statistical Significance Important
Reaching statistical significance can be challenging, especially if you have pages with exceptionally low traffic or changes that are too insignificant to generate results.
Here are some common challenges to reaching statistical significance:
- Not enough time to run tests
- Pages with exceptionally low traffic
- Changes are too insignificant to generate results
To overcome these challenges, you can try running tests on pages that get more traffic or making larger changes in your tests. This can help you achieve statistical significance faster.
Using Unbalanced Traffic
Using unbalanced traffic can increase the chances of your campaign failing or generating inconclusive results. This is because A/B testing should be done with the appropriate traffic to get significant results.
A/B testing with lower or higher traffic than required can lead to unreliable results. Businesses often make this mistake, which can be frustrating and time-consuming.
Testing with the right amount of traffic ensures that your results are accurate and reliable. This helps you make data-driven decisions that improve your website and increase conversions.
Achieving the same results over and over again reduces the chance that your initial result was a fluke. This is why repeatability is crucial in A/B testing.
Testing too many changes at the same time can also lead to inaccurate results. Instead, test more frequently to improve accuracy and scale your efforts.
Worth a look: Why Is Lead Generation Important
What Are the Types?
There are four basic types of A/B testing: A/B testing, Split URL testing, Multivariate testing, and Multipage testing.
A/B testing is perfect for small changes, like changing a button color on a landing page, and can be used to determine which version performs better.
Split URL testing is ideal for testing entirely new page designs, where you can create a separate URL and funnel your experimental group to it.
Multivariate testing is helpful for confirming or negating assumptions about what asset will perform best, and can include multiple variables like different CTA sizes, headlines, and images.
Each additional variable in multivariate testing adds another version of the asset that needs to be created, which can be time-consuming.
Multipage testing is used to gauge the success of an alternative version of workflows or funnels, and can involve sweeping changes to multiple pages in a sequence.
Here are the four types of A/B testing in a nutshell:
Each type of testing has its own advantages and disadvantages, and the choice of which one to use depends on the specific goals and requirements of your project.
Multivariate
Multivariate testing is a more complex form of testing that involves changes to multiple variations of page elements implemented and tested at the same time. This approach allows testers to collect data on which combination of changes performs best.
Multivariate testing can save time and resources by providing useful conclusions in a shorter period. It eliminates the need to run multiple A/B tests on the same web page when the goals of each change are similar.
The total number of versions in a multivariate test can be calculated using the formula: [No. of variations of element A] x [No. of variations of element B] x [No. of variations of element C]… = [Total No. of variations]. This can help you save time, money, and effort and come to a conclusion in the shortest possible time.
Multivariate testing typically offers three primary benefits: it helps avoid the need to conduct several sequential A/B tests with the same goal, easily analyzes and determines the contribution of each page element to the measured gains, and maps all the interactions between all independent element variations.
A fresh viewpoint: How Important Is Hdmi 2.1 for Series X
A multivariate test can be complex and may require more traffic to achieve statistical significance, which can make it take longer to achieve reliable results. However, the benefits of multivariate testing can be significant, making it a valuable tool for advanced marketing, product, and development professionals.
Here's an example of how multivariate testing can be used: Let's say you want to test 2 versions of the hero image, 2 versions of the call-to-action button color, and 2 versions of the headlines of one of your landing pages. This means a total of 8 variations are created, which will be concurrently tested to find the winning variation.
Frequently Asked Questions
What is the significance test for AB test?
Statistical significance in AB testing measures the likelihood that observed differences between versions are real, not due to error or chance. A higher significance level (e.g. 95%) means you're more confident that results are not random.
Featured Images: pexels.com