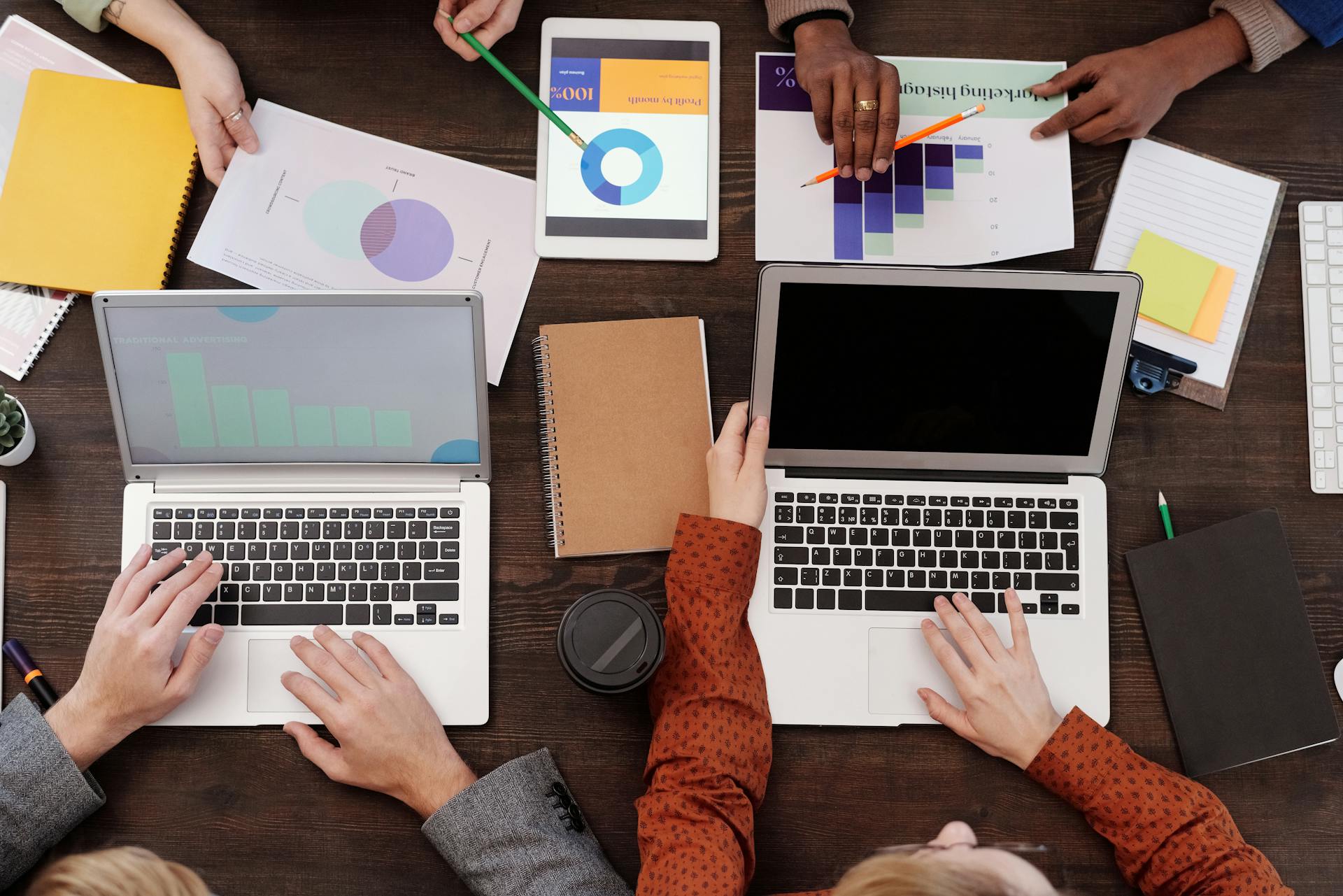
Skewness is a crucial concept in statistics that helps us understand the shape of a distribution, which is essential for making accurate predictions.
Skewness measures the asymmetry of a distribution, with a positive skew indicating a longer tail on the right side and a negative skew indicating a longer tail on the left side.
Understanding skewness is important because it can affect the results of statistical analysis, such as regression and hypothesis testing.
Skewness can also impact the accuracy of predictions, as a skewed distribution can lead to biased or misleading results.
What Is Skewness?
Skewness is a measure of the symmetry of your data distribution. It's used to determine if your data is evenly distributed or if it's leaning towards one side.
A symmetrical distribution is one where the data looks the same to the left and right of the center point. This is the case with a normal distribution, where the mean, median, and mode are all the same.
For more insights, see: One Important Purpose of a Brand Is to
Skewness can be either positive or negative, and it's indicated by the direction of the tail. A negative skewness means the left tail is long relative to the right tail, while a positive skewness means the right tail is long relative to the left tail.
In a symmetrical distribution, the mean, median, and mode are all equal. But in a skewed distribution, these values can be different. For example, in a positively skewed distribution, the mean is greater than the median, which is greater than the mode.
The skewness formula, known as the Fisher-Pearson Coefficient, is the third standardized moment around the mean. It can help you determine the direction and degree of skewness in your data.
Skewness can be caused by outliers or data that has a natural lower bound. For instance, time can't be less than zero without a time machine, which can cause skewness in data related to time.
Broaden your view: How Long Should You Keep Important Papers
Importance of Skewness
Understanding skewness is crucial because it helps explain your process.
Skewness is a reflection of your process, which is why it's essential to grasp its significance.
Your data is a mirror of your process, and skewness can reveal underlying issues that need attention.
Since your data is a reflection of your process, understanding the reasons for skewness will help explain your process.
Curious to learn more? Check out: Why Is Reflection Important
Measures of Skewness
Measures of Skewness are numerous, and each has its own strengths and weaknesses. One type of measure is the Quantile-based measure, which calculates the difference between the average of the upper and lower quartiles and the median.
These measures are often easy to interpret but can show larger sample variations than moment-based methods, leading to misleading results in some cases. For example, samples from a symmetric distribution, like the uniform distribution, can have a large Quantile-based skewness just by chance.
Pearson's moment coefficient of skewness is another measure, which is a multiple of the nonparametric skew. This measure is useful for symmetric distributions, but it can be biased for non-normal distributions.
Measures of Central Tendency
Measures of Central Tendency are important when dealing with skewed data, and understanding their relationship with skewness is crucial.
The mean is a common measure of central tendency, but it can be distorted by skewness, making the median a better choice in some cases.
In a perfectly symmetrical distribution, the mean, median, and mode are all equal, but this is not always the case.
A rule of thumb states that the mean is right of the median under right skew and left of the median under left skew, but this rule fails frequently, especially in multimodal distributions or discrete distributions where the areas to the left and right of the median are not equal.
If your data is skewed, using the median as a measure of central tendency may be a better choice, especially when making predictions or projections.
The medcouple is a robust measure of skewness that is scale-invariant and has a breakdown point of 25%.
Consider reading: Why Is Median Important
Here's a quick comparison of the mean, median, and mode in different distributions:
Understanding the relationship between measures of central tendency and skewness is essential when working with data.
Easy to Compute
Computing skewness is a breeze, thanks to most computer programs that can do the math for you.
The closer your skewness value is to zero, the more symmetrical your data is.
You can easily spot skewness in a histogram, which provides a visual picture of your data and highlights any skewness that's present.
A negative skewness value indicates left skewness, while a positive value points to right skewness.
This makes it easy to identify the direction of your skewness and look for explanations in that area.
Intriguing read: Why Is Customer Lifetime Value Important
Pearson's First Coefficient
Pearson's first skewness coefficient, also known as mode skewness, is a measure of skewness that's been used in statistics.
This measure is defined as the difference between the mode and the mean, divided by the standard deviation.
It's worth noting that this measure is different from Pearson's moment coefficient of skewness, which is another measure of skewness.
A different take: Why Airplane Mode Is Important
Pearson's Median Coefficient
Pearson's median coefficient is a measure of skewness that's worth knowing about. It's defined as a simple multiple of the nonparametric skew. This coefficient is closely related to Kelly's measure of skewness.
The Pearson median coefficient is a useful alternative to the mean when the distribution is too skewed. This is because the mean can be distorted by skewness, making it a less reliable measure of central tendency.
Pearson's second skewness coefficient, also known as the Pearson median skewness, is defined as a simple multiple of the nonparametric skew. This coefficient is a straightforward way to quantify skewness in a distribution.
Groeneveld and Meeden's coefficient is another measure of skewness that's closely related to Pearson's second skewness coefficient. It's defined as the median minus the mean, with the absolute value of the difference.
Worth a look: When Communicating It's Important to
Sample Size and Sampling Precision
Sample size and sampling precision are crucial aspects of statistical analysis, and skewness plays a significant role in determining the necessary sample size to meet specifications for closeness and confidence.
The necessary sample size changes depending on the level of skewness in the distribution. For example, as the skewness parameter λ deviates from zero, the distribution becomes increasingly skewed.
In a normal distribution, where λ is zero, the interval around the location parameter is symmetrical. However, when the distribution is skewed, the interval around the location parameter is not symmetrical.
The necessary sample size to meet specifications for closeness and confidence is not easily calculated and requires numerical methods. Fortunately, the necessary equations for obtaining sample sizes can be derived, as shown in Appendix A.
Using these equations, it's possible to determine the necessary sample sizes to meet various specifications for closeness and confidence, given the level of skewness in the distribution. For instance, if the closeness or precision is set at 0.1, 0.2, 0.3, 0.4, or 0.5, and the confidence level is set at 0.95 or 0.90, the necessary sample size can be calculated.
The level of skewness has a significant impact on the necessary sample size. For example, as the skewness increases from 0 to 5.0, the necessary sample size increases significantly. Figures 2 and 3 illustrate this relationship between skewness and sample size.
In general, it's essential to consider the level of skewness in the distribution when determining the necessary sample size to meet specifications for closeness and confidence.
For more insights, see: Why Is Zero Trust Important
Data Distribution and Skewness
Data distribution and skewness are closely related concepts in statistics. Skewness helps you understand how your data is distributed.
Not all distributions should be assumed to be symmetrical, and skewness will help you understand this.
A distribution may be skewed as a result of an outlier, so it's essential to determine if that's the cause of the skewness.
Discover more: The Most Important Aspect S of a Company's Business Strategy
Data Insights
Skewness can indicate that something is off with your data, so it's essential to review it to understand the cause.
You may need to take action if you find something unexplained or unexpected in your data.
The mean can be distorted by skewness, which is why it's not always the best measure of central tendency.
In such cases, the median might be a better choice, as it's less affected by extreme values.
A single outlier can skew a distribution, making it look asymmetrical.
You should investigate whether that outlier is the cause of the skewness, as it might be a one-time error.
If the skewness is due to an outlier, you might have a symmetrical distribution after all.
In some cases, skewness might be a natural condition of the data, while in others it could be caused by a special factor like outliers or a multi-modal distribution.
You should try to determine whether the skewness is a natural part of the data or if there's a specific reason behind it.
Data Distribution
Skewness is a key concept in understanding how your data is distributed. It's not always symmetrical, and that's where skewness comes in.
A normal distribution has two parameters: the mean and the standard deviation. But for skewed data, we need to add an extra parameter, which is called the skew-normal family.
The skew-normal family includes distributions that are not symmetrical, and it's characterized by a skewness parameter λ. This parameter determines how skewed the distribution is.
In fact, the skew-normal family has a unique probability density function (pdf) that's different from the normal distribution. The pdf is given by f(z) = 2ϕ(z)Φ(λz), where ϕ(·) and Φ(·) are the pdf and cumulative distribution function (cdf) of the standard normal distribution, respectively.
A unique perspective: Why Standard Is Important
Understanding skewness is crucial in data analysis because it can help you identify patterns and anomalies in your data. By recognizing skewness, you can choose the right statistical methods to analyze your data accurately.
Skewness can be either positive or negative, and it affects the shape of the distribution. As skewness deviates from zero, the distribution becomes narrower and more peaked.
Here's a summary of the skew-normal family:
The skew-normal family provides a flexible way to model skewed data, and it's widely used in many fields, including finance, engineering, and social sciences. By understanding skewness and the skew-normal family, you can gain insights into your data and make more informed decisions.
Applications and Use Cases
Skewness is a descriptive statistic that can be used in conjunction with the histogram and the normal quantile plot to characterize the data or distribution. It indicates the direction and relative magnitude of a distribution's deviation from the normal distribution.
Skewness can be used to obtain approximate probabilities and quantiles of distributions via the Cornish–Fisher expansion. This is particularly useful in finance for calculating value at risk.
Understanding skewness is crucial when many models assume normal distribution, which has a skewness of zero. If data points are not perfectly symmetric, an understanding of skewness indicates whether deviations from the mean are going to be positive or negative.
Use for Prediction
Using averages for prediction can be misleading if your data is skewed, and the median might be a better choice in such cases.
The average may not accurately represent the central tendency of skewed data, which can lead to inaccurate predictions.
If you take corrective action to revert to a symmetrical distribution, using the average might be a good option.
Skewed data can result in poor predictions, but identifying and addressing the cause of skewness can help you make more accurate projections.
Take a look at this: Which of the following Is Important When Using Technology
An Industry Example
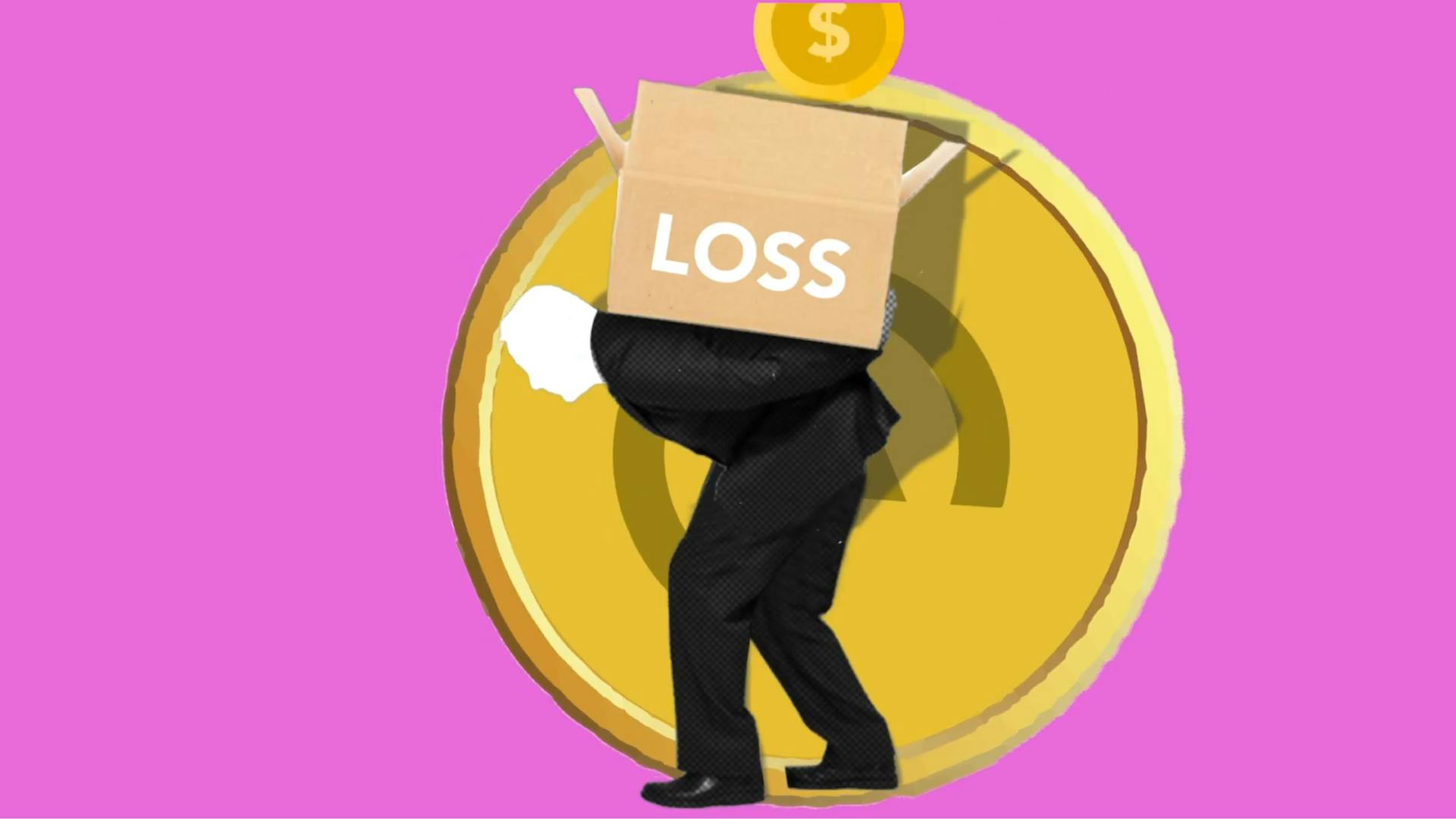
In an office building, the facilities manager was reviewing maintenance records for eight elevators and noticed an average downtime of 3 hours per elevator.
The manager was concerned about the inconvenience this could be causing tenants and was considering firing the elevator maintenance company.
A staff member suggested creating a histogram of the data to look deeper into the situation.
The histogram revealed that the average downtime was skewed by unusually high downtimes due to a lack of replacement parts.
The median downtime of 1.75 hours was a more accurate representation of the elevators' performance.
By stocking more replacement parts, the facilities manager was able to eliminate longer downtimes and improve overall elevator performance.
Intriguing read: Why Is Slack Important to the Project Manager
Frequently Asked Questions
What is the objective of skewness in statistics?
The main objective of skewness in statistics is to gain a complete understanding of a dataset's shape, direction, and form, as well as its symmetry. This helps to provide a more comprehensive view of the population being studied.
Sources
- https://openstax.org/books/introductory-business-statistics-2e/pages/2-6-skewness-and-the-mean-median-and-mode
- https://www.simplilearn.com/tutorials/statistics-tutorial/skewness-and-kurtosis
- https://en.wikipedia.org/wiki/Skewness
- https://pmc.ncbi.nlm.nih.gov/articles/PMC6318746/
- https://www.isixsigma.com/dictionary/skewness/
Featured Images: pexels.com